A Speaker Recognition Method Based on Multi-Stream Hierarchical Fusion Transform Features and Long Short-Term Memory Networks
A long-short-term memory and speaker identification technology, which is applied in the field of speaker identification, can solve the problems of poor identification results, failure to obtain better results, and inability to effectively describe the differences in deep-seated characteristics of speakers.
- Summary
- Abstract
- Description
- Claims
- Application Information
AI Technical Summary
Problems solved by technology
Method used
Image
Examples
Embodiment
[0079] Such as figure 1 Shown is the flow chart of the embodiment of the present invention, and concrete steps are as follows:
[0080] S1. Acoustic feature extraction: Extracting Filterbank features and MFCC features from speech samples, specifically including the following steps:
[0081] S1.1. Pre-emphasis: f(z)=1-αz -1 Filter the input speech for the transfer function, where the value range of α is [0.9,1];
[0082] S1.2. Framing: After pre-emphasis, the voice is divided into voice frames of a specific length, and the frame length is L, the frame shift is S, and the voice of the rth frame is expressed as x r (n), where 1≤r≤R, 0≤n≤N-1, R and N represent the number of frames and the sampling points of each frame of speech respectively;
[0083] S1.3. Windowing: Multiply each frame of speech by the window function w(n), and the window function is a Hamming window, which is recorded as:
[0084]
[0085] S1.4. Extracting Filterbank features and MFCC features, the specif...
PUM
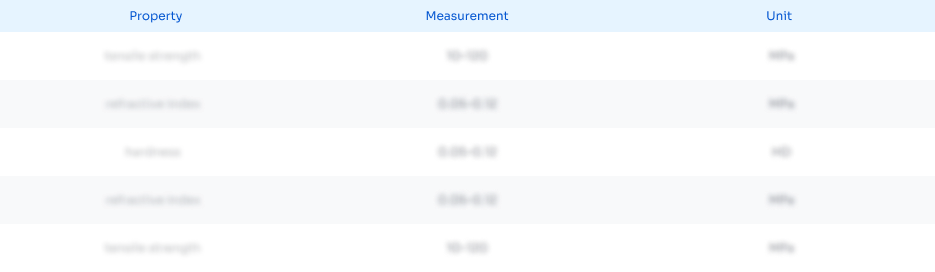
Abstract
Description
Claims
Application Information
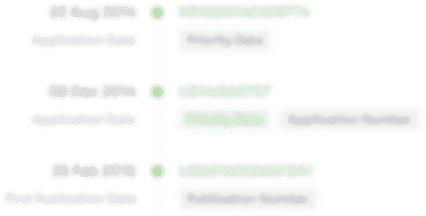
- Generate Ideas
- Intellectual Property
- Life Sciences
- Materials
- Tech Scout
- Unparalleled Data Quality
- Higher Quality Content
- 60% Fewer Hallucinations
Browse by: Latest US Patents, China's latest patents, Technical Efficacy Thesaurus, Application Domain, Technology Topic, Popular Technical Reports.
© 2025 PatSnap. All rights reserved.Legal|Privacy policy|Modern Slavery Act Transparency Statement|Sitemap|About US| Contact US: help@patsnap.com