Image fusion method based on depth learning
An image fusion and deep learning technology, applied in the field of image fusion based on deep learning, can solve the problems of manual definition of filters, difficulty in obtaining prior knowledge, and reduction of dependence on prior knowledge.
- Summary
- Abstract
- Description
- Claims
- Application Information
AI Technical Summary
Problems solved by technology
Method used
Image
Examples
Embodiment Construction
[0023] A method for image fusion based on deep learning, comprising the following steps:
[0024] 1. Construct the basic unit of deep stacked convolutional neural network
[0025] The deep stacked convolutional neural network is composed of multiple basic units stacked. The basic unit is composed of a high-frequency subnetwork, a low-frequency subnetwork and a fusion convolutional layer. The high-frequency subnetwork and low-frequency subnetwork are respectively composed of three convolutional layers. , where the first convolutional layer restricts the input information, the second convolutional layer combines the information, and the third convolutional layer combines the information into high-frequency and low-frequency feature maps, specifically as follows:
[0026] (1) Input the source image x, and get the feature map of the first convolutional layer H1 of the high-frequency subnetwork through convolution operation In the formula, Represents the convolution operation,...
PUM
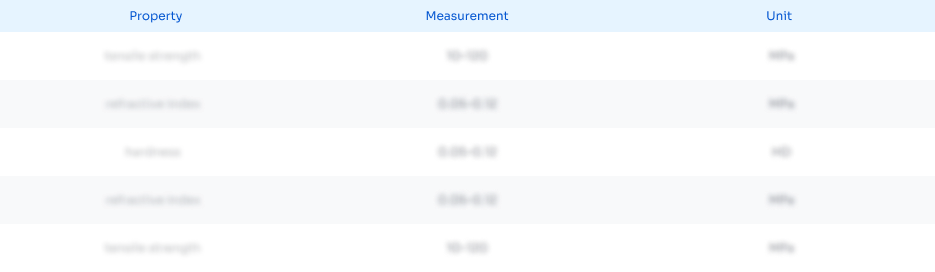
Abstract
Description
Claims
Application Information
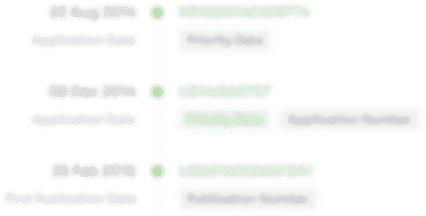
- Generate Ideas
- Intellectual Property
- Life Sciences
- Materials
- Tech Scout
- Unparalleled Data Quality
- Higher Quality Content
- 60% Fewer Hallucinations
Browse by: Latest US Patents, China's latest patents, Technical Efficacy Thesaurus, Application Domain, Technology Topic, Popular Technical Reports.
© 2025 PatSnap. All rights reserved.Legal|Privacy policy|Modern Slavery Act Transparency Statement|Sitemap|About US| Contact US: help@patsnap.com