Medical image classification method based on collaborative deep learning
A medical image and classification method technology, applied in the field of medical image classification based on collaborative deep learning, can solve problems such as poor classification accuracy, achieve the effect of strengthening feature representation, feature learning ability, and overcoming similar problems between classes
- Summary
- Abstract
- Description
- Claims
- Application Information
AI Technical Summary
Problems solved by technology
Method used
Image
Examples
Embodiment Construction
[0023] The specific steps of the medical image classification method based on collaborative deep learning in the present invention are as follows:
[0024] 1. Image pair input.
[0025] Using the input method of image pairs, two images are randomly sampled from the training images and input into two corresponding convolutional neural networks for training. Each pair of images has three supervision signals, which are the respective category labels of the two images and whether the pair of images belong to the same category. These three signals jointly supervise the training of the model.
[0026] 2. Dual deep convolutional neural network training.
[0027] The dual deep convolutional neural network module is the basic component of this algorithm, which includes two complete convolutional neural networks A and B with independent functions. In principle, convolutional neural networks of any structure can be applied to this algorithm module by deep feature extraction based on de...
PUM
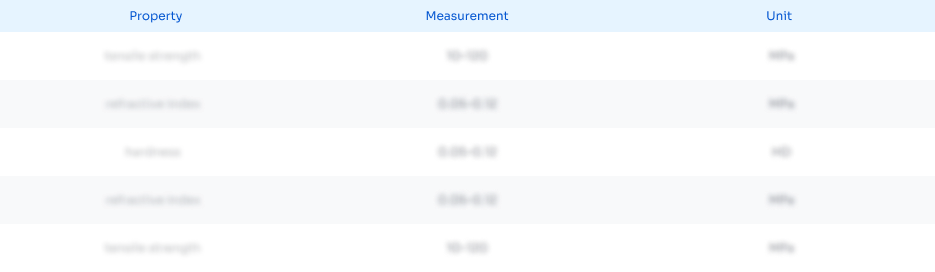
Abstract
Description
Claims
Application Information
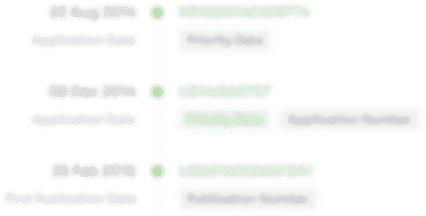
- R&D Engineer
- R&D Manager
- IP Professional
- Industry Leading Data Capabilities
- Powerful AI technology
- Patent DNA Extraction
Browse by: Latest US Patents, China's latest patents, Technical Efficacy Thesaurus, Application Domain, Technology Topic, Popular Technical Reports.
© 2024 PatSnap. All rights reserved.Legal|Privacy policy|Modern Slavery Act Transparency Statement|Sitemap|About US| Contact US: help@patsnap.com