End-to-end weak supervision target detection method based on salient guidance
A technology of target detection and weak supervision, applied in the direction of instruments, character and pattern recognition, computer components, etc., can solve the problems of no mining and little improvement in target detection performance
- Summary
- Abstract
- Description
- Claims
- Application Information
AI Technical Summary
Problems solved by technology
Method used
Image
Examples
Embodiment Construction
[0064] The present invention is further described below.
[0065] Embodiments of the present invention and its implementation process are:
[0066] (1) Collect an image I with a known image-level label, and the image-level label is y=[y 1 ,y 2 ,...,y C ], where y c Represents the label corresponding to the c-th category object in the image, the label is divided into foreground label and background label, each label attribute is foreground label or background label, y c ∈{1,-1},y c =1 means that there is an object of the cth category in the image, y c =-1 means that there is no c-th category object in the image, one label corresponds to one category object, and C is the total number of category objects;
[0067] (2) Process the image I to obtain the category-related saliency map M corresponding to each category object c , candidate target area and with each candidate target region set of adjacent superpixels;
[0068] Use the DCSM algorithm to process the image I to...
PUM
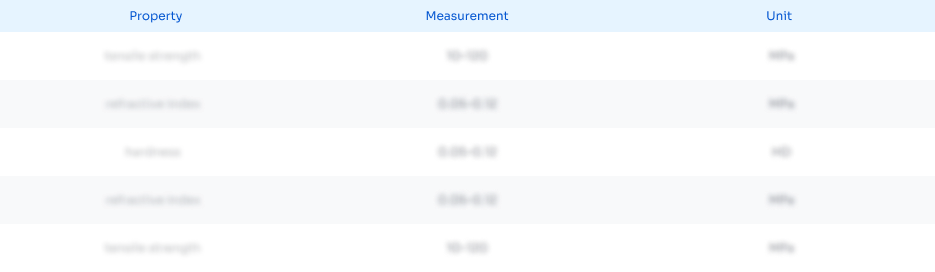
Abstract
Description
Claims
Application Information
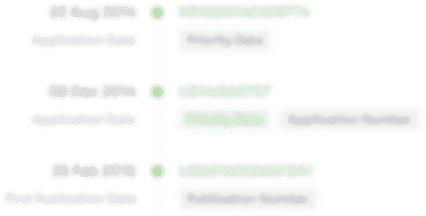
- R&D Engineer
- R&D Manager
- IP Professional
- Industry Leading Data Capabilities
- Powerful AI technology
- Patent DNA Extraction
Browse by: Latest US Patents, China's latest patents, Technical Efficacy Thesaurus, Application Domain, Technology Topic, Popular Technical Reports.
© 2024 PatSnap. All rights reserved.Legal|Privacy policy|Modern Slavery Act Transparency Statement|Sitemap|About US| Contact US: help@patsnap.com