Multi-classifier-based convolutional neural network quick classification method
A convolutional neural network, fast classification technology, applied in neural learning methods, biological neural network models, neural architectures, etc., can solve problems such as lack of computing power
- Summary
- Abstract
- Description
- Claims
- Application Information
AI Technical Summary
Problems solved by technology
Method used
Image
Examples
Embodiment Construction
[0020] The specific implementation manners of the present invention will be further described in detail below in conjunction with the accompanying drawings.
[0021] like figure 1 As shown, the network training method mainly includes determining the number of additional classifiers and training all classifiers at the same time. Proceed as follows:
[0022] S1. Construct a standard convolutional neural network (CNN), including N convolutional layers and 1 classifier. Using the standard image dataset D train (such as the MNIST handwritten data set, CIFAR-10 data set, etc., the number of samples is set to 1) to train the network, and obtain the feature vector (vector, V) of the image after each layer of convolution (not including the last convolution layer). N-1) and single-sample time consumption (γ orginal , that is, the time consumption required for the sample to go from the input layer to the output of the classifier). The training relies on back propagation (BP), and th...
PUM
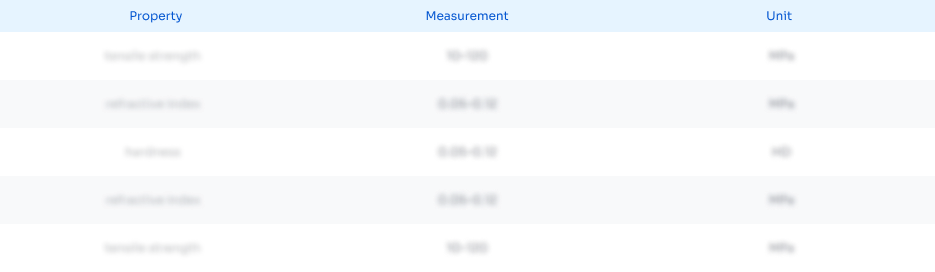
Abstract
Description
Claims
Application Information
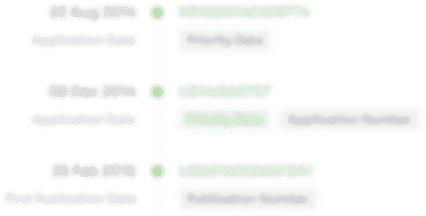
- R&D Engineer
- R&D Manager
- IP Professional
- Industry Leading Data Capabilities
- Powerful AI technology
- Patent DNA Extraction
Browse by: Latest US Patents, China's latest patents, Technical Efficacy Thesaurus, Application Domain, Technology Topic, Popular Technical Reports.
© 2024 PatSnap. All rights reserved.Legal|Privacy policy|Modern Slavery Act Transparency Statement|Sitemap|About US| Contact US: help@patsnap.com