A transfer learning method for high spatial resolution remote sensing images based on deep features
A technology with high spatial resolution and depth features, applied in instruments, scene recognition, computing, etc., can solve the problem of inability to use remote sensing images, improve the effect of image transfer learning, and avoid differences in image spectral values.
- Summary
- Abstract
- Description
- Claims
- Application Information
AI Technical Summary
Problems solved by technology
Method used
Image
Examples
Embodiment Construction
[0025] An example of implementation of a transfer learning method for high spatial resolution remote sensing images based on depth features using the present invention is attached figure 1 As shown, the attached figure 1 Describe it.
[0026]The processing unit 100 performs principal component transformation on current common multi-band high-spatial-resolution remote sensing images (such as four-band IKONOS satellite multispectral images, eight-band WorldView-4 satellite multispectral images), using the first three components ( That is, the first three components with the most information) generate a three-band image.
[0027] The processing unit 101 reads an image block of an odd window size (such as 5×5, 7×7, 9×9, 11×11) with the geometric center of each pixel of the image obtained in the processing unit 100, the image A block acts as a representation that the pixel has spatial context information.
[0028] The processing unit 102 inputs the existing convolutional neura...
PUM
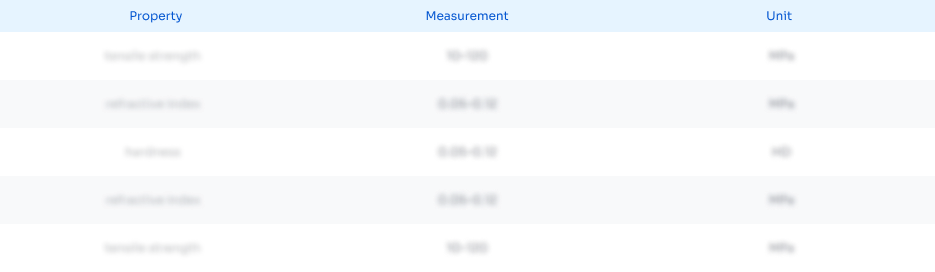
Abstract
Description
Claims
Application Information
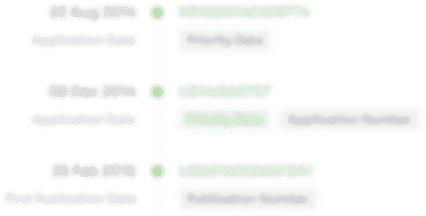
- R&D
- Intellectual Property
- Life Sciences
- Materials
- Tech Scout
- Unparalleled Data Quality
- Higher Quality Content
- 60% Fewer Hallucinations
Browse by: Latest US Patents, China's latest patents, Technical Efficacy Thesaurus, Application Domain, Technology Topic, Popular Technical Reports.
© 2025 PatSnap. All rights reserved.Legal|Privacy policy|Modern Slavery Act Transparency Statement|Sitemap|About US| Contact US: help@patsnap.com