Adaptive soft measurement prediction method based on Bayesian network with sliding window
A Bayesian network and sliding window technology, applied in the field of adaptive soft measurement prediction based on Bayesian network with sliding window, can solve the problems of model no longer applicable to running state, model degradation, etc. Prediction, the effect of high prediction accuracy
- Summary
- Abstract
- Description
- Claims
- Application Information
AI Technical Summary
Problems solved by technology
Method used
Image
Examples
Embodiment Construction
[0022] The present invention is aimed at the soft measurement problem in the industrial process. The method first selects the data closest to the sample to be predicted from the data set as the training sample, uses the method of Bayesian network to build a model, and uses the data of the sample to be predicted Inputs are added to the network as evidence, and predictions are made after inference. When the new samples accumulate to a certain amount, delete the old samples, add new samples, update the training sample set, and re-establish the Bayesian network for the prediction of subsequent new samples. The specific technical scheme is as follows:
[0023] An adaptive soft sensor prediction method based on a Bayesian network with a sliding window, comprising the following steps:
[0024] Step 1: Collect historical data sets in industrial processes: take easy-to-measure process variables as input, that is, X=[x 1 ; x 2 ;…;x n ]∈R n×m , where each column of X represents a pro...
PUM
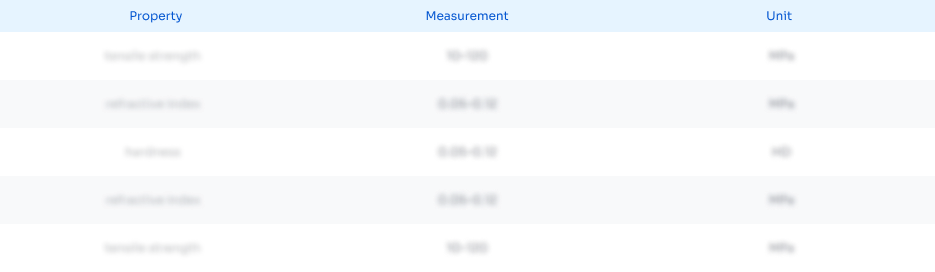
Abstract
Description
Claims
Application Information
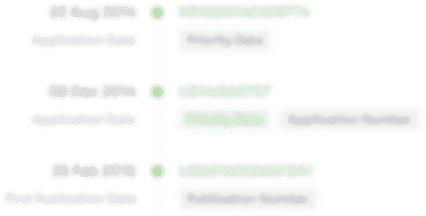
- R&D
- Intellectual Property
- Life Sciences
- Materials
- Tech Scout
- Unparalleled Data Quality
- Higher Quality Content
- 60% Fewer Hallucinations
Browse by: Latest US Patents, China's latest patents, Technical Efficacy Thesaurus, Application Domain, Technology Topic, Popular Technical Reports.
© 2025 PatSnap. All rights reserved.Legal|Privacy policy|Modern Slavery Act Transparency Statement|Sitemap|About US| Contact US: help@patsnap.com