Image de-noising method based on compression convolutional neural network
A convolutional neural network and image technology, applied in the field of digital images, can solve problems such as multi-space cost, and achieve the effect of compressing the number of network layers, excellent denoising effect, and excellent denoising effect
- Summary
- Abstract
- Description
- Claims
- Application Information
AI Technical Summary
Problems solved by technology
Method used
Image
Examples
Embodiment Construction
[0031]The technical solution of the present invention will be described in detail below with reference to the drawings and embodiments.
[0032] like image 3 As shown, an image denoising method based on a compressed denoising convolutional neural network disclosed in an embodiment of the present invention mainly includes: 1. Constructing a training data set; 2. Constructing a compressed denoising convolution based on low-rank matrix decomposition 3. Use the training data set to train the network model; 4. After training, input the image with noise into the network, output the noise image with the same size as the original image, and then convert the image polluted by noise Subtracting this output image yields the denoised image.
[0033] The constructed compressed denoising convolutional neural network reduces the number of layers of the original denoising neural network DnCNN. The original DnCNN has two networks for different purposes, and their structures are basically th...
PUM
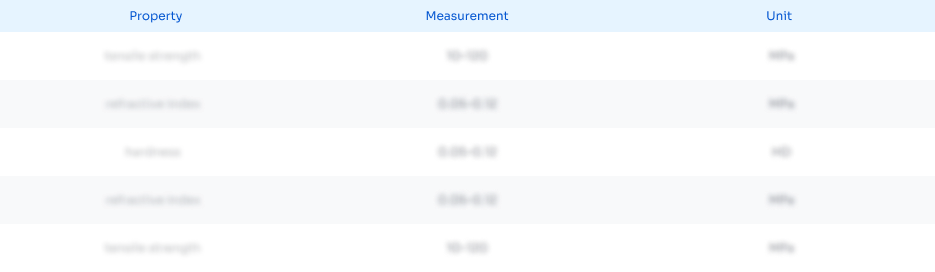
Abstract
Description
Claims
Application Information
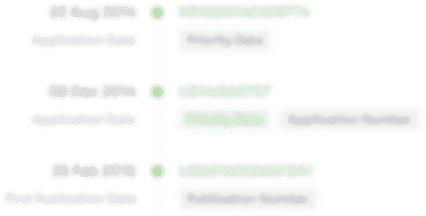
- R&D Engineer
- R&D Manager
- IP Professional
- Industry Leading Data Capabilities
- Powerful AI technology
- Patent DNA Extraction
Browse by: Latest US Patents, China's latest patents, Technical Efficacy Thesaurus, Application Domain, Technology Topic.
© 2024 PatSnap. All rights reserved.Legal|Privacy policy|Modern Slavery Act Transparency Statement|Sitemap