Fabric defect detection and classification method based on unsupervised segmentation and ELM (Extreme Learning Machines)
A classification method and defect detection technology, applied in character and pattern recognition, instruments, computer components, etc., can solve the problems of unsatisfactory classification accuracy and cumbersome training process of classifiers to achieve good segmentation effect and cumbersome training process , the effect of improving the accuracy rate
- Summary
- Abstract
- Description
- Claims
- Application Information
AI Technical Summary
Problems solved by technology
Method used
Image
Examples
Embodiment 1
[0027] Embodiment 1: as Figure 1-8 Shown, a fabric defect detection and classification method based on unsupervised segmentation and ELM. First, input the fabric defect image, realize the segmentation of the fabric defect image, and obtain the segmented fabric defect image; secondly, extract the shape and texture features of the segmented fabric defect image and the original defect image, and realize the feature extraction of the fabric defect image; then classify The fabric defect image feature set and label are used as the training set to train the ELM classifier to obtain the parameters of the ELM classifier; finally, the trained classifier is fused according to the Bayesian probability to realize the classification of the fabric defect image and output the classification result of the fabric defect image . The detection and classification method adopted in the present invention has a higher accuracy rate.
[0028] The concrete steps of described method are as follows: ...
Embodiment 2
[0034] Embodiment 2: as figure 1 As shown, the specific steps of the method are as follows: Step1, input fabric defect image G ' , by extracting the image G ' patch {x i} Create data matrix X=[x 1 ,x 2 ,...,x i ,...,x n ],x i ∈ R w , w represents the image G ' patch x i Dimensions, n represents the image G ' patch x i The total number, that is, X contains n column vectors whose dimension is w; based on Euclidean distance E(i)=||x i -x|| 2 , where x is {x i}, eliminate the outliers of the data matrix X to obtain the training data, X c =[x 1 ,x 2 ,...,x i ,...,x c ], 1≤i≤ci ∈ R w , w represents the image G ' patch x i Dimensions, where c represents the training data X c Medium image G ' patch x i Total, i.e. X c Contains c column vectors of dimension w; find a dictionary D that represents the training data X with the least squared error c For each point in , by non-convex function formula (a i is each x of dimension k i coefficient vector), by assum...
PUM
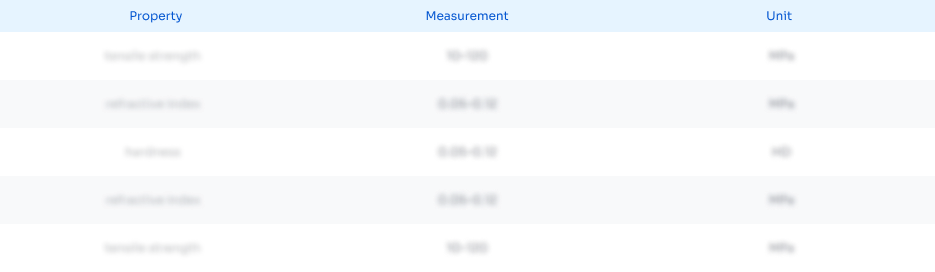
Abstract
Description
Claims
Application Information
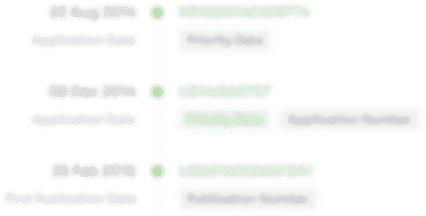
- R&D Engineer
- R&D Manager
- IP Professional
- Industry Leading Data Capabilities
- Powerful AI technology
- Patent DNA Extraction
Browse by: Latest US Patents, China's latest patents, Technical Efficacy Thesaurus, Application Domain, Technology Topic, Popular Technical Reports.
© 2024 PatSnap. All rights reserved.Legal|Privacy policy|Modern Slavery Act Transparency Statement|Sitemap|About US| Contact US: help@patsnap.com