Personalized recommendation method based on dynamic neighboring point spectral clustering
A recommendation method and adjacent point technology, applied in the field of recommendation, can solve problems such as difficult extraction of network features, difficulty in determining the clustering center of clustering algorithms, and inability to implement user clusters to recommend store clusters, etc.
- Summary
- Abstract
- Description
- Claims
- Application Information
AI Technical Summary
Problems solved by technology
Method used
Image
Examples
Embodiment Construction
[0087] The present invention will be further described below in conjunction with the accompanying drawings.
[0088] refer to Figure 1 to Figure 4 , a personalized recommendation method based on dynamic neighborhood spectral clustering, including the following steps:
[0089] 1) Establish a bipartite network based on the user-store based on the check-in data of the database, and map it to two different vector spaces for representation. The process is as follows:
[0090] 1.1) First, a user-store bipartite network is established based on the check-in data in the database, and the weight between the user and the store in the bipartite network is the number of times the user has visited the store;
[0091] 1.2) Unilaterally project the current user-store bipartite network to the user-user network and store-store network respectively, where the weight between user points in the user-user network of the unilateral network is the user The number of the same store that has been vi...
PUM
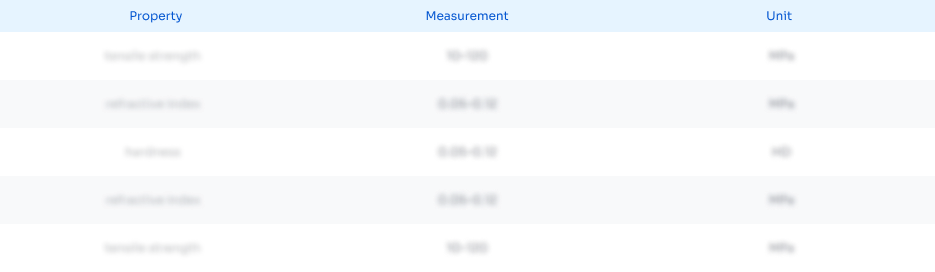
Abstract
Description
Claims
Application Information
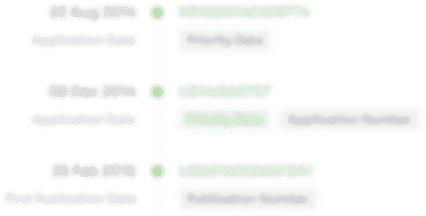
- R&D Engineer
- R&D Manager
- IP Professional
- Industry Leading Data Capabilities
- Powerful AI technology
- Patent DNA Extraction
Browse by: Latest US Patents, China's latest patents, Technical Efficacy Thesaurus, Application Domain, Technology Topic, Popular Technical Reports.
© 2024 PatSnap. All rights reserved.Legal|Privacy policy|Modern Slavery Act Transparency Statement|Sitemap|About US| Contact US: help@patsnap.com