Electric power system short period load prediction method based on combined neural network
A short-term load forecasting and power system technology, applied in neural learning methods, biological neural network models, forecasting, etc., can solve problems such as difficulties in accurate forecasting
- Summary
- Abstract
- Description
- Claims
- Application Information
AI Technical Summary
Problems solved by technology
Method used
Image
Examples
Embodiment Construction
[0052] In conjunction with the accompanying drawings, the technical solution will be further described.
[0053] Step 1: Collect and summarize the data collection and summary of power grid load data and meteorological data in historical areas, and import them into the Excel database.
[0054] Step 2: Data preprocessing. In order to avoid the occurrence of neuron saturation, it is necessary to preprocess the original load data. This will help the convergence of the training process and improve the prediction accuracy. The main preprocessing method is to count the maximum and minimum values of the historical load data in the training sample set, and normalize the load data to the [-1, 1] interval, which can make the data at the same level and speed up the convergence of the neural network .
[0055] Step 3: Determine the model structure.
[0056] The generalization performance of a single NN in different sample input spaces is different, which will affect the prediction accu...
PUM
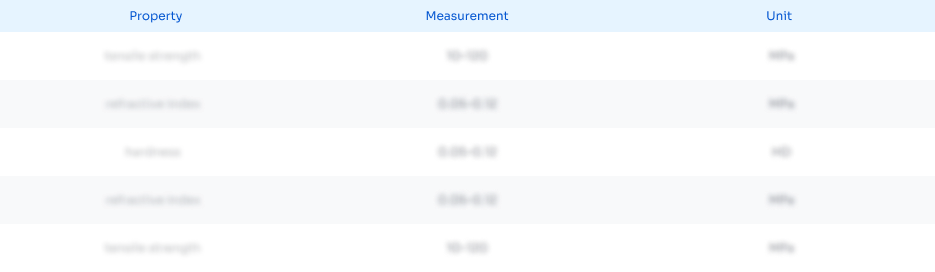
Abstract
Description
Claims
Application Information
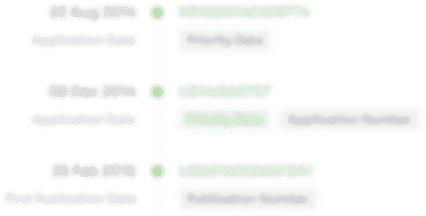
- R&D Engineer
- R&D Manager
- IP Professional
- Industry Leading Data Capabilities
- Powerful AI technology
- Patent DNA Extraction
Browse by: Latest US Patents, China's latest patents, Technical Efficacy Thesaurus, Application Domain, Technology Topic.
© 2024 PatSnap. All rights reserved.Legal|Privacy policy|Modern Slavery Act Transparency Statement|Sitemap