Convolutional neural network and active learning-based ceramic tile surface defect recognition method
A convolutional neural network, active learning technology, applied in character and pattern recognition, image data processing, instruments, etc., can solve the problem of high time complexity, achieve the effect of accelerating convergence and reducing labeling costs
- Summary
- Abstract
- Description
- Claims
- Application Information
AI Technical Summary
Problems solved by technology
Method used
Image
Examples
Embodiment Construction
[0038] The present invention provides a ceramic tile surface defect detection method based on convolutional neural network and active learning. In order to further illustrate the technical solution of the present invention, examples are given below in conjunction with the accompanying drawings. It should be understood that the examples given here are only used to explain the present invention, not to limit the present invention.
[0039] Such as figure 1 As shown, a tile surface defect detection method based on convolutional neural network and active learning includes the following steps:
[0040] (1) Obtain images and preprocess: acquire surface images of tiles with defects, the number should not be less than 1000, and perform preprocessing.
[0041] (2) The establishment of the training set: the preprocessed tile surface image obtains more image blocks through the sliding window method, and its set is U, which is divided into 5 parts equally, that is, U={U 1 ,U 2 ,U 3 ,U...
PUM
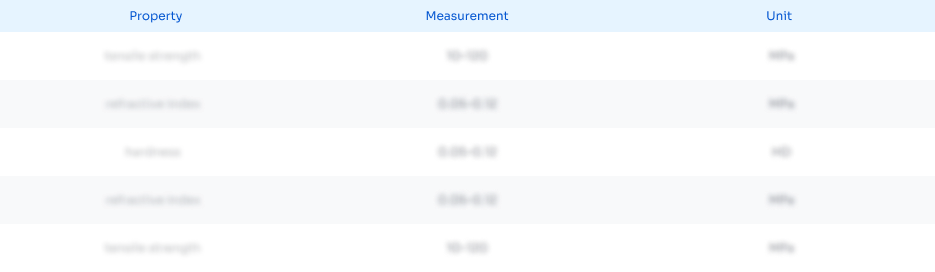
Abstract
Description
Claims
Application Information
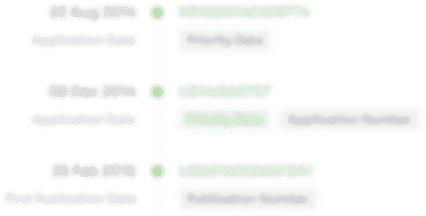
- R&D Engineer
- R&D Manager
- IP Professional
- Industry Leading Data Capabilities
- Powerful AI technology
- Patent DNA Extraction
Browse by: Latest US Patents, China's latest patents, Technical Efficacy Thesaurus, Application Domain, Technology Topic.
© 2024 PatSnap. All rights reserved.Legal|Privacy policy|Modern Slavery Act Transparency Statement|Sitemap