End-to-end difference network learning method for image semantic segmentation
A technology of semantic segmentation and network learning, applied in the field of image processing, it can solve the problems of mutual occlusion and illumination changes, blurred edges, and inability to obtain accurate edges of the target, and achieve the effect of high segmentation quality and improved segmentation performance.
- Summary
- Abstract
- Description
- Claims
- Application Information
AI Technical Summary
Problems solved by technology
Method used
Image
Examples
Embodiment Construction
[0039] The technical solutions and beneficial effects of the present invention will be described in detail below in conjunction with the accompanying drawings.
[0040] like figure 1 As shown, the present invention provides an end-to-end difference network learning method for image semantic segmentation, comprising the following steps:
[0041] Step 1, build the network structure: Use the Caffe deep learning framework to build the main network structure and the complete network structure respectively, where the main network structure is used to generate the rough segmentation model and the small target area of each image in the training set, and the complete network is used for the final image semantic segmentation;
[0042] Step 2, main network model training: use part of the data in the training set to train the rough model of the main network, and compare the segmentation result obtained by the rough model with the real segmentation map to obtain the misclassified area o...
PUM
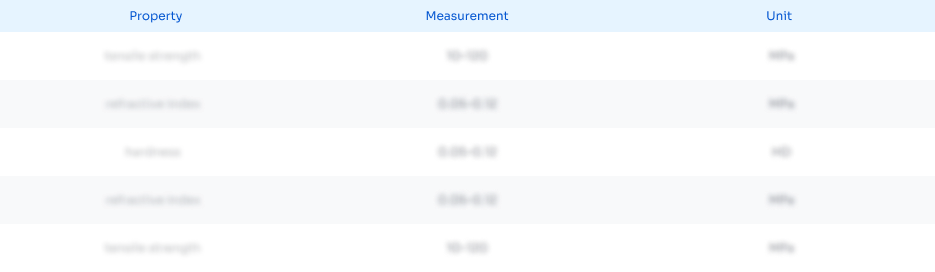
Abstract
Description
Claims
Application Information
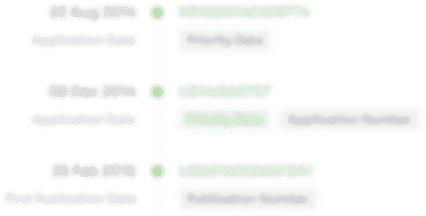
- R&D
- Intellectual Property
- Life Sciences
- Materials
- Tech Scout
- Unparalleled Data Quality
- Higher Quality Content
- 60% Fewer Hallucinations
Browse by: Latest US Patents, China's latest patents, Technical Efficacy Thesaurus, Application Domain, Technology Topic, Popular Technical Reports.
© 2025 PatSnap. All rights reserved.Legal|Privacy policy|Modern Slavery Act Transparency Statement|Sitemap|About US| Contact US: help@patsnap.com