Image emotion classification method based on ResNet-GCN network
An emotion classification and image technology, applied in neural learning methods, biological neural network models, instruments, etc., can solve the problems of increasing network complexity, lack of specific analysis, and reducing network generalization ability, so as to achieve comprehensive and increase the emotional characteristics of images. The effect of learning vision and reducing bias
- Summary
- Abstract
- Description
- Claims
- Application Information
AI Technical Summary
Problems solved by technology
Method used
Image
Examples
Embodiment
[0054] Such as figure 1 As shown, the image emotion classification method based on the ResNet-GCN network of this implementation includes the following steps:
[0055] (1) The structural design of the image emotion classification network, the specific network parameter settings and methods are as follows:
[0056] Such as figure 2 As shown, the image emotion classification network ResNet-GCN of the present invention consists of two parts, the front part and the back part. The previous part of the structure borrowed ResNet-50 [K.He, X. Zhang, S. Ren, et al, Deep Residual Learning for Image Recognition, IEEE Conference on Computer Vision and Pattern Recognition (CVPR), pp: 770-778, 2016. ] structure and parameters. This part contains 5 convolutional layer groups, 1 fully connected layer and 1 Softmax layer.
[0057] Such as image 3 As shown, the latter part of the structure borrows GCN [Peng C, Zhang X, Yu G, et al. Large KernelMatters—Improve Semantic Segmentation by Glo...
PUM
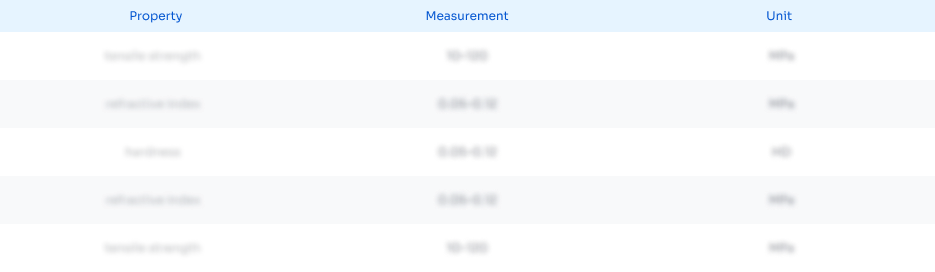
Abstract
Description
Claims
Application Information
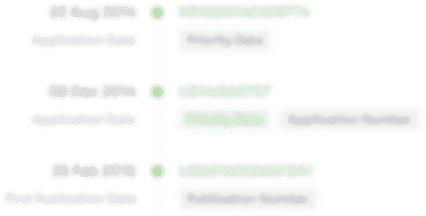
- R&D
- Intellectual Property
- Life Sciences
- Materials
- Tech Scout
- Unparalleled Data Quality
- Higher Quality Content
- 60% Fewer Hallucinations
Browse by: Latest US Patents, China's latest patents, Technical Efficacy Thesaurus, Application Domain, Technology Topic, Popular Technical Reports.
© 2025 PatSnap. All rights reserved.Legal|Privacy policy|Modern Slavery Act Transparency Statement|Sitemap|About US| Contact US: help@patsnap.com