Object Detection Method of Optical Remote Sensing Image Based on Dense Object Feature Learning
A technology of optical remote sensing images and target features, which is applied in the field of optical remote sensing image target detection based on dense target feature learning, can solve the problems of insufficient utilization of multi-scale feature target information, low detection accuracy of dense small targets, and low detection effect of dense small targets Unsatisfactory problems, to achieve the effect of improving accuracy and ensuring accuracy
- Summary
- Abstract
- Description
- Claims
- Application Information
AI Technical Summary
Problems solved by technology
Method used
Image
Examples
Embodiment Construction
[0037] The present invention will be further described in detail below with reference to the accompanying drawings.
[0038] Refer to the attached figure 1 , the steps of the present invention are described in further detail.
[0039] Step 1. Build a 25-layer dense target feature network and set the parameters of each layer of the network.
[0040] The structure of the dense target feature network is: input layer→first convolutional layer→second convolutional layer→first pooling layer→third convolutional layer→fourth convolutional layer→ The second pooling layer → the fifth convolutional layer → the sixth convolutional layer → the seventh convolutional layer → the third pooling layer → the eighth convolutional layer → the ninth convolutional layer → the first Ten convolutional layers → fourth pooling layer → eleventh convolutional layer → twelfth convolutional layer → thirteenth convolutional layer → first upsampling layer → RPN classification and regression layer → ROI Pooli...
PUM
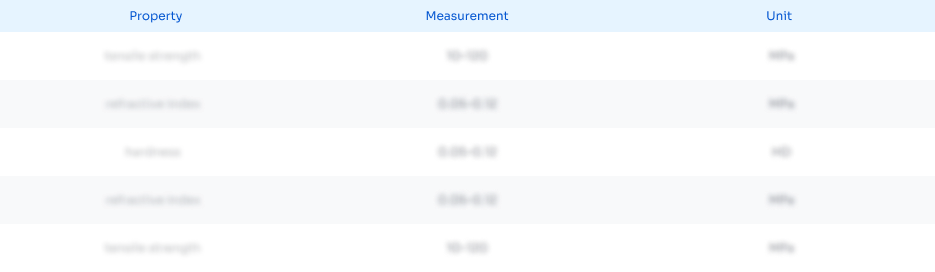
Abstract
Description
Claims
Application Information
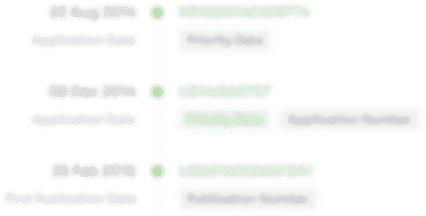
- R&D Engineer
- R&D Manager
- IP Professional
- Industry Leading Data Capabilities
- Powerful AI technology
- Patent DNA Extraction
Browse by: Latest US Patents, China's latest patents, Technical Efficacy Thesaurus, Application Domain, Technology Topic, Popular Technical Reports.
© 2024 PatSnap. All rights reserved.Legal|Privacy policy|Modern Slavery Act Transparency Statement|Sitemap|About US| Contact US: help@patsnap.com