Data balancing strategy and multi-feature fusion-based image labeling method
A multi-feature fusion and image annotation technology, which is applied in the fields of electrical digital data processing, special data processing applications, character and pattern recognition, etc., can solve problems such as weak expressive ability of a single feature feature and unbalanced training image set
- Summary
- Abstract
- Description
- Claims
- Application Information
AI Technical Summary
Problems solved by technology
Method used
Image
Examples
Embodiment Construction
[0036] In order to make the present invention more comprehensible, a preferred embodiment is now described in detail with accompanying drawings as follows.
[0037] like figure 1 As shown, a data balancing strategy and a multi-feature fusion image labeling method of the present invention, the method first adopts the data balancing strategy to expand the semantic group of image training, and input it into the trained deep convolutional neural network , the image is abstracted into a deep feature vector through multiple iterations of convolution and downsampling, and the features of each semantic group image are obtained; then the multi-scale fusion features of each semantic group are calculated; and it is combined with the deep convolutional neural network Multi-feature fusion is performed on the deep features calculated by the network; a complete feature representation of the semantic group is obtained. Use the same method to extract the features of the image to be labeled an...
PUM
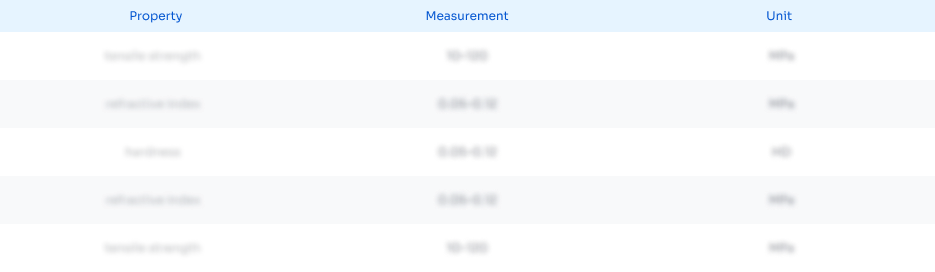
Abstract
Description
Claims
Application Information
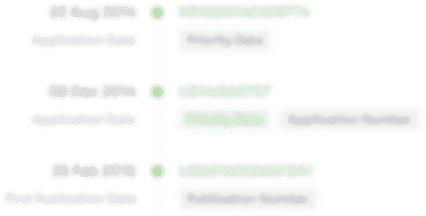
- R&D Engineer
- R&D Manager
- IP Professional
- Industry Leading Data Capabilities
- Powerful AI technology
- Patent DNA Extraction
Browse by: Latest US Patents, China's latest patents, Technical Efficacy Thesaurus, Application Domain, Technology Topic, Popular Technical Reports.
© 2024 PatSnap. All rights reserved.Legal|Privacy policy|Modern Slavery Act Transparency Statement|Sitemap|About US| Contact US: help@patsnap.com