A Weld Defect Recognition Method Based on Improved Lenet-5 Model
A defect recognition and model technology, applied in the field of weld defect recognition, can solve problems such as manual selection, achieve the effect of comprehensive image features and enhanced feature extraction capabilities
- Summary
- Abstract
- Description
- Claims
- Application Information
AI Technical Summary
Problems solved by technology
Method used
Image
Examples
Embodiment Construction
[0038] The present invention provides a weld defect recognition method based on the improved LeNet-5 model, and improves the input of the traditional convolution kernel channel of the LeNet-5 model for the gray image of the weld, that is, the gray image is enhanced by false color technology, convert it into a color image, and use the obtained color image as the input of the neural network; improve the convolution kernel of the LeNet-5 model, add a convolution channel with a Gabor filter, and in the sixth layer of the neural network, the The features obtained by multiple channels are fused; the SoftMax multi-class classifier is used in the output layer to obtain the probability that the weld defect belongs to each category.
[0039] see figure 1 , the present invention a kind of weld defect recognition method based on improved LeNet-5 model, comprises the following steps:
[0040] S1. For the grayscale image of the weld, the input of the traditional convolution kernel channel ...
PUM
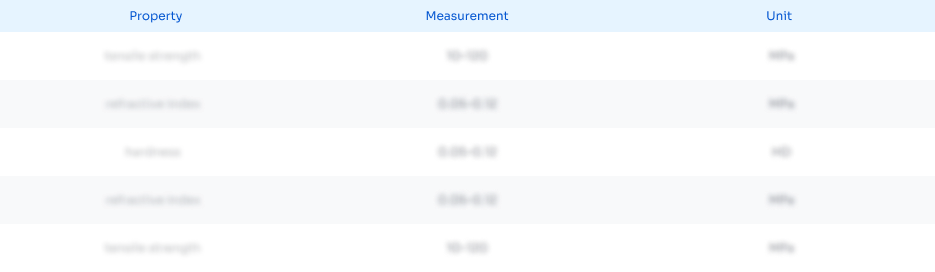
Abstract
Description
Claims
Application Information
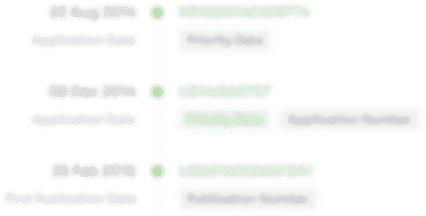
- R&D
- Intellectual Property
- Life Sciences
- Materials
- Tech Scout
- Unparalleled Data Quality
- Higher Quality Content
- 60% Fewer Hallucinations
Browse by: Latest US Patents, China's latest patents, Technical Efficacy Thesaurus, Application Domain, Technology Topic, Popular Technical Reports.
© 2025 PatSnap. All rights reserved.Legal|Privacy policy|Modern Slavery Act Transparency Statement|Sitemap|About US| Contact US: help@patsnap.com