Segmentation loss-based generative adversarial network method
A network and generator technology, applied in the field of deep learning neural network, can solve the problems of poor discriminator feature and robust discriminator feature, and achieve the effect of feature robustness, stable training process, and improvement of mode collapse phenomenon.
- Summary
- Abstract
- Description
- Claims
- Application Information
AI Technical Summary
Problems solved by technology
Method used
Image
Examples
Embodiment Construction
[0035] Machine learning algorithms can be divided into supervised learning with labels and unsupervised learning without labels. Due to the high cost of obtaining labeled data for supervised learning and the lack of performance of unsupervised learning algorithms, semi-supervised learning (SSL) has become an important research direction for researchers. SSL can learn robust features by using a large number of unlabeled samples and a small number of labeled samples, and has a good performance in image classification. Lee et al. proposed an efficient method of pseudo-labeling unlabeled data to help model training. Rasmus et al. proposed a ladder network based on an autoencoder. The encoder is used for supervised learning, and each layer of the decoder corresponds to the encoder one-to-one to form a ladder for unsupervised learning training.
[0036] In recent years, Deep generative models (DGMs) and Generative Adversarial Networks (GAN) have performed well in semi-supervised le...
PUM
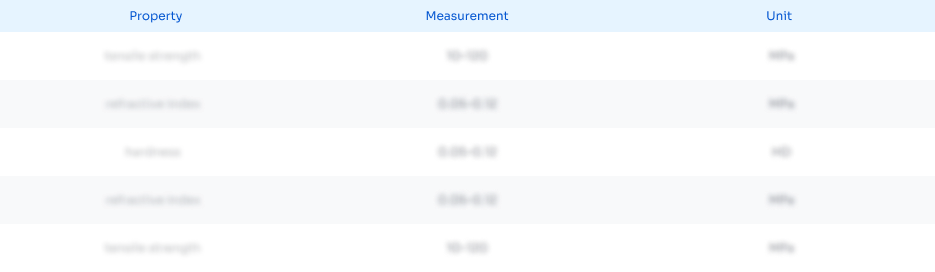
Abstract
Description
Claims
Application Information
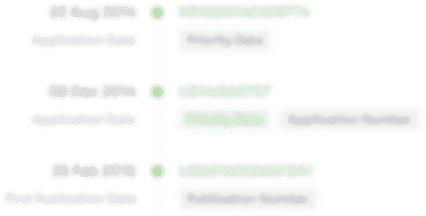
- R&D Engineer
- R&D Manager
- IP Professional
- Industry Leading Data Capabilities
- Powerful AI technology
- Patent DNA Extraction
Browse by: Latest US Patents, China's latest patents, Technical Efficacy Thesaurus, Application Domain, Technology Topic.
© 2024 PatSnap. All rights reserved.Legal|Privacy policy|Modern Slavery Act Transparency Statement|Sitemap