Class-Centric Vector Text Classification Based on Dependency Relationship, Speech and Semantic Dictionary
A technology of dependency relationship and semantic dictionary, which is applied in the field of class center vector text classification, can solve the problems of long classification time, low classification efficiency, and low classification accuracy, and achieve the effect of improving classification effect, improving classification accuracy, and solving feature loss
- Summary
- Abstract
- Description
- Claims
- Application Information
AI Technical Summary
Problems solved by technology
Method used
Image
Examples
Embodiment 1
[0092] Experimental comparison of feature selection
[0093] This example combines the three-layer feature selection of dependency relationship, semantic dictionary and part-of-speech to obtain F as shown in Table 3. 1 The comparison results of value improvement.
[0094] Table 3 Feature selection pair F 1 value increase
[0095]
[0096]
[0097] As can be seen from Table 3, when the feature selection is only based on the dependency relationship, the classification experiments of Bayesian, KNN and the text classification method of the present invention on the Fudan corpus, Sogou corpus and 20Newsgroups corpus respectively show that based on the dependency relationship. The feature selection method has a very good classification effect; after introducing the semantic dictionary based on the feature selection method based on the dependency relationship, compared with the traditional feature selection, the improvement range is between 1.52% and 7.91%, and the contributio...
Embodiment 2
[0099] Comparison of improved experiments of class center vector method
[0100] According to the class center vector text classification method based on dependency relationship, part of speech and semantic dictionary proposed by the present invention, the present invention has carried out experiment respectively on three corpus, aim at three innovations of the method of the present invention, and original class center vector The method was compared experimentally, as shown in Table 4.
[0101] Table 4 The improved method of the present invention and the comparison result of the traditional class center vector method
[0102]
[0103] It can be seen from Table 4 that the improved method of the present invention and the class center vector method have carried out three stages of comparative experiments. The F1 values of the three stages have been improved to varying degrees, and the time spent is getting shorter and shorter. This is mainly due to the fact that the present...
Embodiment 3
[0105] Experimental comparison of classification efficiency of class center vector method
[0106] There are many text classification algorithms, such as Bayesian algorithm, KNN algorithm, and class center vector method. Using Bayesian, KNN and class center vector method to conduct ten cross-validation classification experiments on the three preprocessed corpus, and count the classification time and use F 1 The experimental results are shown in Table 5.
[0107] Table 5 Comparison of classification algorithm efficiency and accuracy
[0108]
[0109] As can be seen from Table 5, in the classification experiments of Fudan corpus, Sogou corpus and 20Newsgroups corpus, the class center vector method of the present invention is the shortest classification method, and other classification algorithms are time-consuming.
PUM
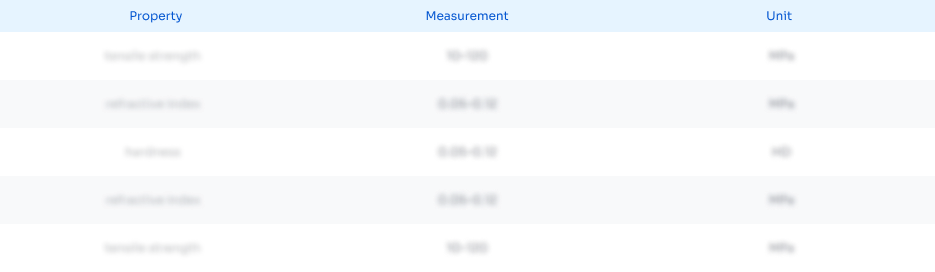
Abstract
Description
Claims
Application Information
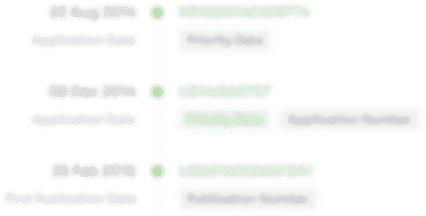
- R&D Engineer
- R&D Manager
- IP Professional
- Industry Leading Data Capabilities
- Powerful AI technology
- Patent DNA Extraction
Browse by: Latest US Patents, China's latest patents, Technical Efficacy Thesaurus, Application Domain, Technology Topic, Popular Technical Reports.
© 2024 PatSnap. All rights reserved.Legal|Privacy policy|Modern Slavery Act Transparency Statement|Sitemap|About US| Contact US: help@patsnap.com