A Traceability Method for Sampling-Constrained Active Learning
An active learning and sampling technology, applied in the field of active learning, can solve problems such as outliers, limited system cognition, and imbalance, and achieve the effect of excellent sampling performance
- Summary
- Abstract
- Description
- Claims
- Application Information
AI Technical Summary
Problems solved by technology
Method used
Image
Examples
Embodiment Construction
[0041] In order to describe the technical content of the present invention more clearly, further description will be given below in conjunction with specific embodiments.
[0042] The invention relates to a source tracing method of sampling limited active learning to evaluate the SLP strength under the false source mechanism in a cyber-physical system. Considering the limited system cognition of the traceability agent, which makes the data labeling ability limited, a traceability method with limited sampling is established; considering that unconstrained random walk will lead to a long traceability period, a network traffic identification model is used to Optimize the traceability strategy; considering that the false traffic generated by the false source mechanism may be much higher or lower than the normal traffic, in order to solve this category imbalance and potential outliers, the uncertainty and sample double Active Learning Strategies Combining Representation and Diversi...
PUM
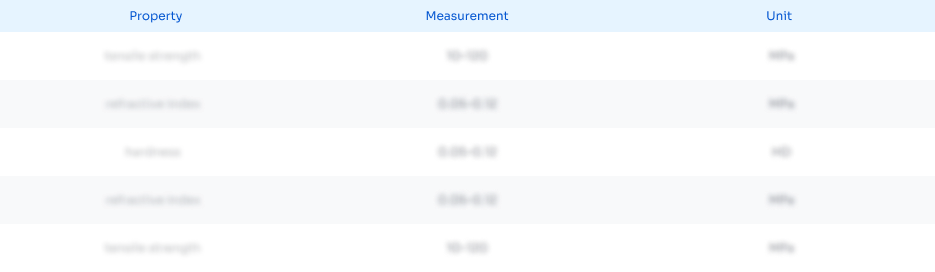
Abstract
Description
Claims
Application Information
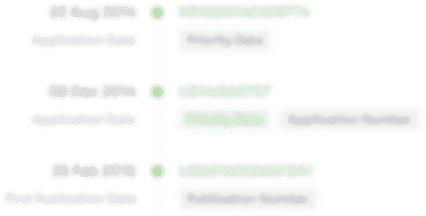
- R&D Engineer
- R&D Manager
- IP Professional
- Industry Leading Data Capabilities
- Powerful AI technology
- Patent DNA Extraction
Browse by: Latest US Patents, China's latest patents, Technical Efficacy Thesaurus, Application Domain, Technology Topic, Popular Technical Reports.
© 2024 PatSnap. All rights reserved.Legal|Privacy policy|Modern Slavery Act Transparency Statement|Sitemap|About US| Contact US: help@patsnap.com