Improved time delay neural network acoustic model
An acoustic model and neural network technology, applied in the field of time-delayed neural network acoustic model, can solve the problems of acoustic model performance to be improved, TDNN model layer features without explicit modeling, etc., to achieve performance improvement and strengthen modeling capabilities Effect
- Summary
- Abstract
- Description
- Claims
- Application Information
AI Technical Summary
Problems solved by technology
Method used
Image
Examples
Embodiment Construction
[0022] The present invention will be further described in detail below in conjunction with the accompanying drawings and specific embodiments.
[0023] An improved time-delay neural network (TDNN) acoustic model, adding a specific module (also known as attention layer, attention layer or attention module) between several hidden layers of TDNN, and using the specific module to process the original input features weighted, and send the weighted features to the next hidden layer.
[0024] The attention module consists of an affine transformation and a weighting function. The output of the previous hidden layer is used as input to extract the feature weight value of the input, and the extracted weight value is used to weight the original input feature (element-by-element multiplication operation), Get the weighted features. The attention module can be effectively combined with TDNN to effectively improve the performance of the TDNN acoustic model without introducing too many para...
PUM
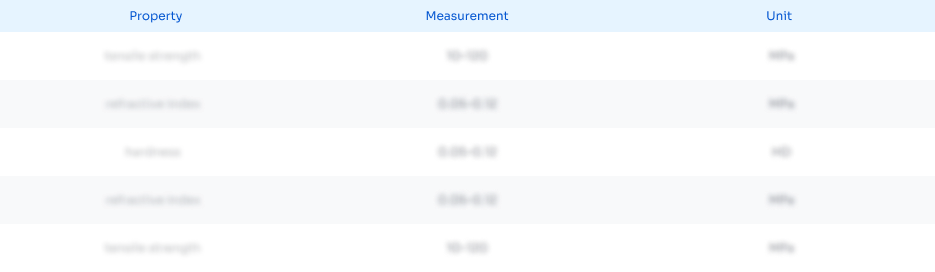
Abstract
Description
Claims
Application Information
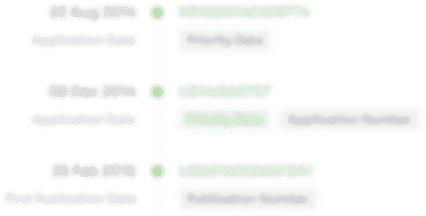
- R&D
- Intellectual Property
- Life Sciences
- Materials
- Tech Scout
- Unparalleled Data Quality
- Higher Quality Content
- 60% Fewer Hallucinations
Browse by: Latest US Patents, China's latest patents, Technical Efficacy Thesaurus, Application Domain, Technology Topic, Popular Technical Reports.
© 2025 PatSnap. All rights reserved.Legal|Privacy policy|Modern Slavery Act Transparency Statement|Sitemap|About US| Contact US: help@patsnap.com