Self-evolutionary radar target detection algorithm based on deep learning
A radar target and deep learning technology, applied in neural learning methods, calculations, computer components, etc., can solve problems such as manual labeling, high cost, and unsuitable data for deep learning models, and achieve reduced manpower consumption and strong practicability , Improve the effect of radar recognition ability
- Summary
- Abstract
- Description
- Claims
- Application Information
AI Technical Summary
Problems solved by technology
Method used
Image
Examples
Embodiment Construction
[0038] In order to make the object, technical scheme and advantages of the present invention clearer, the present invention is further described in detail below to the present invention's embodiment:
[0039] The present invention comprises the following steps:
[0040] 1) The feature processing of radar data, the specific steps are as follows:
[0041] Step 1: In order to facilitate accurate feature learning for deep learning, feature processing is performed on the collected radar data, and spatial features, time features, and motion features are added respectively; firstly, a 3 ×3 area, all the information in the area is formed into an area information set, and at the same time, the relevant indicators of the central area of the detection point are calculated in conjunction with the background information, and the relevant indicators include fluctuation values, SNR values and amplitude values;
[0042] Step 2: In order to be more conducive to deep model learning, the co...
PUM
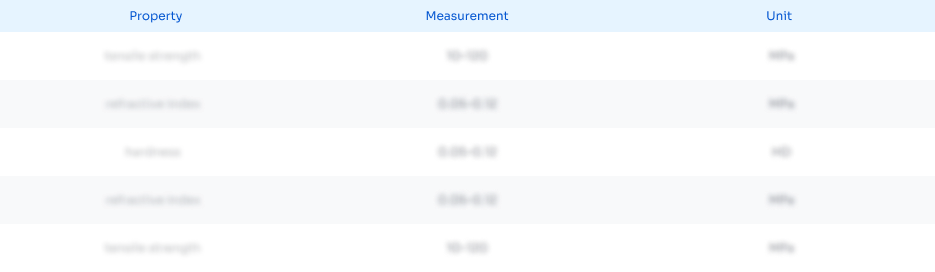
Abstract
Description
Claims
Application Information
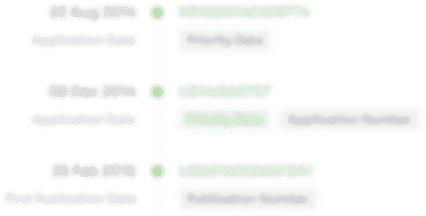
- R&D
- Intellectual Property
- Life Sciences
- Materials
- Tech Scout
- Unparalleled Data Quality
- Higher Quality Content
- 60% Fewer Hallucinations
Browse by: Latest US Patents, China's latest patents, Technical Efficacy Thesaurus, Application Domain, Technology Topic, Popular Technical Reports.
© 2025 PatSnap. All rights reserved.Legal|Privacy policy|Modern Slavery Act Transparency Statement|Sitemap|About US| Contact US: help@patsnap.com