Distributed clustering method of visual objects based on time series
A distributed clustering and time series technology, applied in the field of image recognition, can solve problems such as low efficiency and complex clustering methods, achieve high efficiency, improve clustering efficiency, and reduce time spent
- Summary
- Abstract
- Description
- Claims
- Application Information
AI Technical Summary
Problems solved by technology
Method used
Image
Examples
Embodiment 1
[0032] DBSCAN (density-based spatial clustering of applications with noise) is a more classic density-based clustering algorithm. The input parameters do not need to include the final number of categories, which is in line with our current scene. Its specific definition is as follows: X is a set of points that need to be clustered, and there are three types of points, core points, density-reachable points, and outlier points. If a point p has minPts points (including point p itself) within the range of distance ε, then p is called the core point, and the points within the range of ε become directly reachable by p. Second, if there exists a path p 1 ,...,p n , by p 1 = p and p n =q, and each p i+1 all by p i directly reachable, then q is said to be reachable by p. Finally, all points that are not reachable by any point are called outliers.
[0033] However, only relying on the above-mentioned DBSCAN cannot complete realistic tasks, because the time complexity of the abov...
Embodiment 2
[0045] The time-series-based distributed clustering method for visual objects proposed in the above-mentioned embodiment 1 is used for the actual algorithm coding test, and the specific process is as follows:
[0046] Step S1, collect pictures of the visual target through the camera. The video information can be obtained by connecting with the camera through the RTSP protocol, and the target detection program can be written through the recognition SDK developed by the laboratory, and the picture information and the time information of the picture can be saved to the server hard disk.
[0047] Step S2, through the feature extraction interface of the recognition SDK in the laboratory, feature extraction can be performed on the picture saved in the previous step. After actual testing, it takes about 3 hours to get all the feature data on 4 1080Ti Nvidia graphics cards for 1 million visual target images. The feature data can be stored in the file in binary form. The feature dimen...
PUM
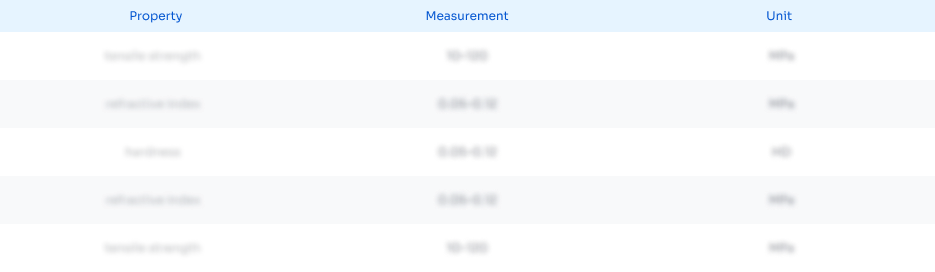
Abstract
Description
Claims
Application Information
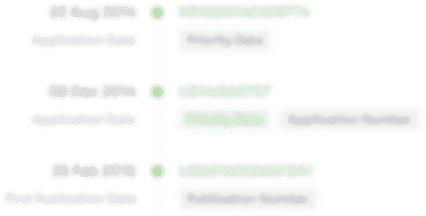
- R&D
- Intellectual Property
- Life Sciences
- Materials
- Tech Scout
- Unparalleled Data Quality
- Higher Quality Content
- 60% Fewer Hallucinations
Browse by: Latest US Patents, China's latest patents, Technical Efficacy Thesaurus, Application Domain, Technology Topic, Popular Technical Reports.
© 2025 PatSnap. All rights reserved.Legal|Privacy policy|Modern Slavery Act Transparency Statement|Sitemap|About US| Contact US: help@patsnap.com