Remote sensing image ground object classification method based on depth learning semantic segmentation network
A technology of semantic segmentation and deep learning, applied in neural learning methods, biological neural network models, image analysis, etc., can solve problems such as tasks that are difficult to support explosive growth and demand workload, and achieve edge classification effects and enhanced features Representational power, the effect of optimizing boundaries
- Summary
- Abstract
- Description
- Claims
- Application Information
AI Technical Summary
Problems solved by technology
Method used
Image
Examples
Embodiment Construction
[0032] Specific embodiments of the present invention will be described below in conjunction with the accompanying drawings, so that those skilled in the art can better understand the present invention. It should be noted that in the following description, when detailed descriptions of known functions and designs may dilute the main content of the present invention, these descriptions will be omitted here.
[0033] figure 1 It is a principle block diagram of a specific implementation of the remote sensing image classification method based on the deep semantic segmentation network of the present invention.
[0034] In this example, if figure 1 The shown remote sensing image object classification method based on deep learning semantic segmentation network includes the following steps:
[0035] 1. Data preparation
[0036] Data preparation includes image collection and labeling, in which high-resolution visible light remote sensing images with different loads are collected, and...
PUM
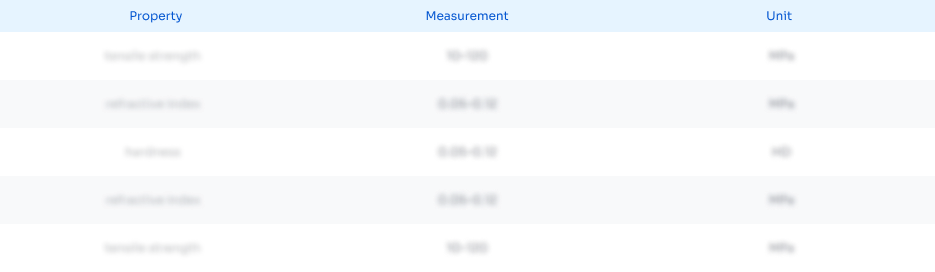
Abstract
Description
Claims
Application Information
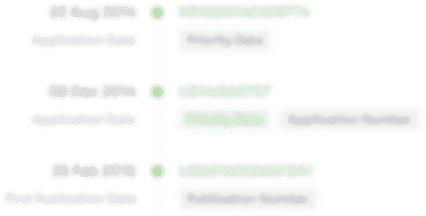
- R&D Engineer
- R&D Manager
- IP Professional
- Industry Leading Data Capabilities
- Powerful AI technology
- Patent DNA Extraction
Browse by: Latest US Patents, China's latest patents, Technical Efficacy Thesaurus, Application Domain, Technology Topic.
© 2024 PatSnap. All rights reserved.Legal|Privacy policy|Modern Slavery Act Transparency Statement|Sitemap