A kind of pathological image classification method and computer equipment
A technology of pathological images and classification methods, applied in image analysis, computer components, computer-aided medical procedures, etc. Fitting and other issues to achieve the effect of improving classification accuracy, realizing classification tasks, and preventing overfitting
- Summary
- Abstract
- Description
- Claims
- Application Information
AI Technical Summary
Problems solved by technology
Method used
Image
Examples
Embodiment 1
[0045] figure 1 A schematic flowchart of the pathological image classification method provided by the first embodiment of the present invention is shown.
[0046] Step S100, performing down-sampling and color transformation on the original image to obtain a first predetermined number of color-enhanced images, and randomly extracting a second predetermined number of sub-images from each of the color-enhanced images;
[0047] As mentioned above, the pathological image is a pathological image of breast cancer;
[0048] Optionally, the size of the original image of breast cancer is 2048×1536 pixels, and the downsampling is performed to reduce its size to half of the original image (1024×768 pixels) as the input image, which avoids the excessive size of the image and leads to pre-training The incompatibility of the network, and allowing the selection of smaller sub-image sizes without causing loss of important feature information; then transforming the original image from RGB spac...
Embodiment 2
[0059] refer to figure 2 , the second embodiment of the present invention provides a pathological image classification method, based on the above figure 1 In the first embodiment shown, the step S200 "extracts the feature vectors of the sub-images respectively through a third predetermined number of deep convolutional neural networks, and extracts the feature vectors of the third predetermined number of deep convolutional neural networks to The eigenvectors of the original image are encoded and fused to obtain the eigenmatrix of the original image, including:
[0060] Step S210, respectively input the sub-images into the first deep convolutional neural network, the second deep convolutional neural network, and the third deep convolutional neural network for image feature extraction to obtain the feature vector;
[0061] As mentioned above, the third predetermined number is three, which are the first deep convolutional neural network, the second deep convolutional neural netw...
Embodiment 3
[0078] refer to image 3 , the third embodiment of the present invention provides a pathological image classification method, based on the above figure 1 In the first embodiment shown, the step S300 of "using the sparse dual-relational regularization feature selection method to perform data dimensionality reduction processing on the feature matrix of the original image to obtain the most relevant feature matrix of the original image" includes:
[0079] S310, according to the feature matrix of the original image and the real label vector of the pathological image, the objective function is optimized by a sparse double-relational regularization feature selection method to obtain a weight coefficient vector, wherein the objective function is expressed as:
[0080]
[0081] where w is the weight coefficient vector; y is the real label vector of the original image;
[0082] Specifically, the objective function optimized by the sparse dual-relational regularization feature selec...
PUM
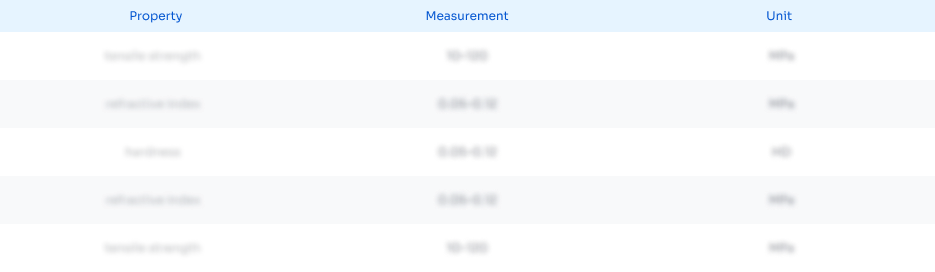
Abstract
Description
Claims
Application Information
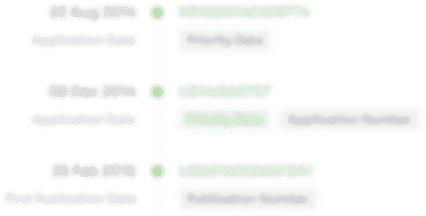
- R&D Engineer
- R&D Manager
- IP Professional
- Industry Leading Data Capabilities
- Powerful AI technology
- Patent DNA Extraction
Browse by: Latest US Patents, China's latest patents, Technical Efficacy Thesaurus, Application Domain, Technology Topic, Popular Technical Reports.
© 2024 PatSnap. All rights reserved.Legal|Privacy policy|Modern Slavery Act Transparency Statement|Sitemap|About US| Contact US: help@patsnap.com