A grey neural simulation method for civil buildings with non-artificial cold and heat sources at room temperature is disclosed
A technology of civil architecture and simulation methods, applied in neural learning methods, design optimization/simulation, biological neural network models, etc., can solve problems such as large prediction errors, unstable performance, and regardless of the impact of system development, and achieve improved accuracy , improve the utilization rate, and improve the effect of simulation accuracy
- Summary
- Abstract
- Description
- Claims
- Application Information
AI Technical Summary
Problems solved by technology
Method used
Image
Examples
Embodiment Construction
[0076] The present invention will be further described below in conjunction with specific examples, but the present invention is not limited to these specific implementations. Those skilled in the art will realize that the present invention covers all alternatives, modifications and equivalents as may be included within the scope of the claims.
[0077] The present invention combines the optimized multi-variable gray prediction OGM (1, N) model with the BP neural network method, and uses measured data and a typical annual meteorological database as the data basis to develop a simulation for the indoor temperature of civil buildings with non-artificial cold and heat sources. method (OGMBPT for short). The training data of OGMBPT adopts the relevant data measured in accordance with the "Civil Building Indoor Heat and Humidity Environment Evaluation Standard" GBT50785-2012. The simulated meteorological parameter set of OGMBPT uses local typical year outdoor meteorological data c...
PUM
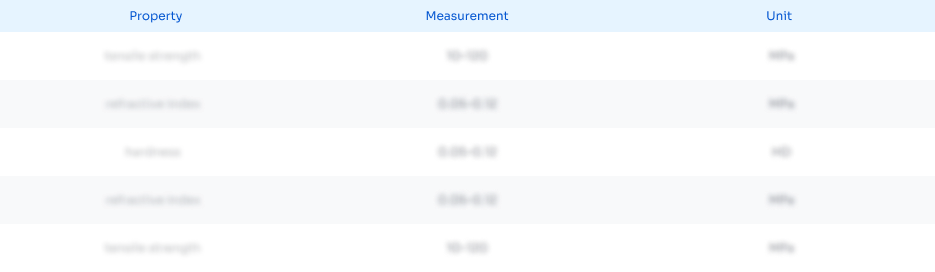
Abstract
Description
Claims
Application Information
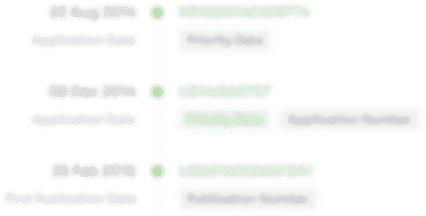
- R&D
- Intellectual Property
- Life Sciences
- Materials
- Tech Scout
- Unparalleled Data Quality
- Higher Quality Content
- 60% Fewer Hallucinations
Browse by: Latest US Patents, China's latest patents, Technical Efficacy Thesaurus, Application Domain, Technology Topic, Popular Technical Reports.
© 2025 PatSnap. All rights reserved.Legal|Privacy policy|Modern Slavery Act Transparency Statement|Sitemap|About US| Contact US: help@patsnap.com