Non-reference image quality evaluation method based on convolutional self-coding network
A convolutional self-encoding and quality evaluation technology, applied in the field of no-reference image quality evaluation, can solve the problems of low image quality sensitivity, no consideration of the integrity of image semantic content, low accuracy of model evaluation results, etc., and achieve accurate results , good results
- Summary
- Abstract
- Description
- Claims
- Application Information
AI Technical Summary
Problems solved by technology
Method used
Image
Examples
Embodiment Construction
[0036] The present invention will be further described below in conjunction with the accompanying drawings and simulation experiments.
[0037] Refer to attached figure 1 , to further describe in detail the specific steps of the present invention.
[0038] Step 1. Build a convolutional autoencoder network.
[0039]Build a 17-layer convolutional autoencoder network and set the parameters of each layer of the convolutional autoencoder network; its structure is as follows: input layer→1st convolutional layer→1st pooling layer→2nd convolutional layer→ 2nd pooling layer→3rd convolutional layer→3rd pooling layer→4th convolutional layer→5th convolutional layer→1st deconvolution layer→2nd deconvolution layer → 1st anti-pooling layer → 3rd deconvolution layer → 2nd anti-pooling layer → 4th deconvolution layer → 4th anti-pooling layer → 5th deconvolution layer;
[0040] Set the parameters of each layer of the convolutional autoencoder network as follows:
[0041] Set the number of c...
PUM
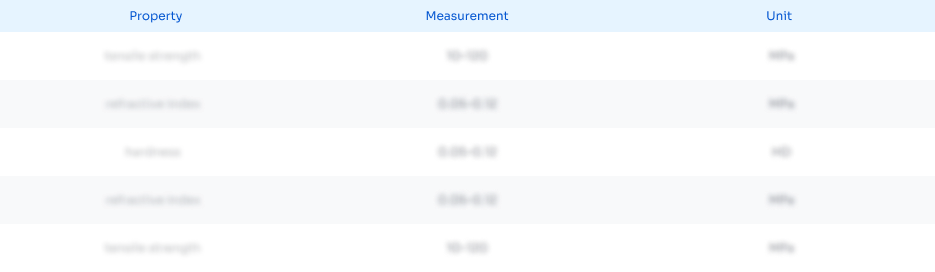
Abstract
Description
Claims
Application Information
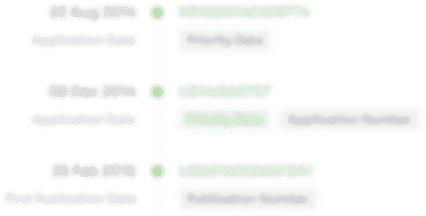
- R&D Engineer
- R&D Manager
- IP Professional
- Industry Leading Data Capabilities
- Powerful AI technology
- Patent DNA Extraction
Browse by: Latest US Patents, China's latest patents, Technical Efficacy Thesaurus, Application Domain, Technology Topic, Popular Technical Reports.
© 2024 PatSnap. All rights reserved.Legal|Privacy policy|Modern Slavery Act Transparency Statement|Sitemap|About US| Contact US: help@patsnap.com