An image semantic segmentation method based on local region conditional random field model
A conditional random field and local area technology, applied in the field of computer vision, can solve the problems of high computational complexity and limited application, and achieve the effect of reducing the computational process, reducing the time complexity, and improving the segmentation accuracy.
- Summary
- Abstract
- Description
- Claims
- Application Information
AI Technical Summary
Problems solved by technology
Method used
Image
Examples
Embodiment Construction
[0032] An image semantic segmentation method based on a local area conditional random field model, its topology mainly includes a fully convolutional neural network structure, a region selection structure, and a local area conditional random field model structure; the fully convolutional neural network structure is used to extract input images feature and obtain a rough segmentation result, and then send the result to the region selection structure; the region selection structure is used to filter the segmentation result map and select the largest circumscribed rectangle of the part of the segmentation result as pedestrians, bicycles, and motor vehicles, These rectangular areas are then fed into the local area conditional random field model; the local area conditional random field model is used to finely optimize the segmentation results of the above rectangular areas.
[0033] The fully convolutional neural network structure is an improved DeepLab v2 structure, obtained by rep...
PUM
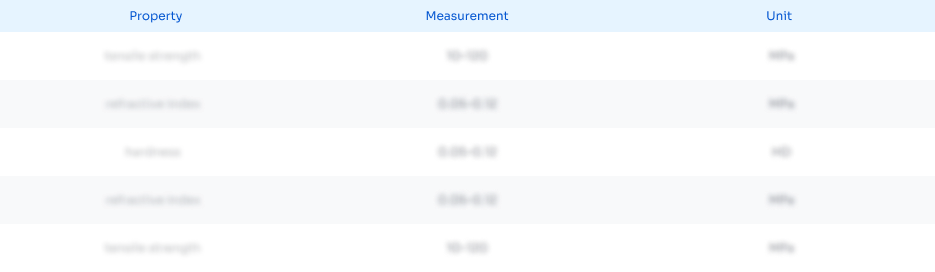
Abstract
Description
Claims
Application Information
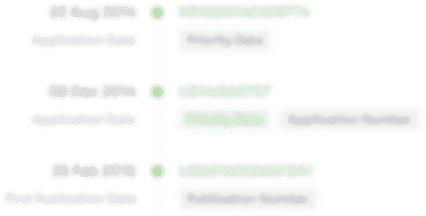
- R&D
- Intellectual Property
- Life Sciences
- Materials
- Tech Scout
- Unparalleled Data Quality
- Higher Quality Content
- 60% Fewer Hallucinations
Browse by: Latest US Patents, China's latest patents, Technical Efficacy Thesaurus, Application Domain, Technology Topic, Popular Technical Reports.
© 2025 PatSnap. All rights reserved.Legal|Privacy policy|Modern Slavery Act Transparency Statement|Sitemap|About US| Contact US: help@patsnap.com