An image classification network training method based on massive single-class and single-amplitude images
A single image and classification network technology, applied in pattern recognition, can solve problems such as unsatisfactory effect, loss of effect of DeepID, no intra-class distance, etc., to increase inter-class distance, reduce intra-class distance, and recognition accuracy high effect
- Summary
- Abstract
- Description
- Claims
- Application Information
AI Technical Summary
Problems solved by technology
Method used
Image
Examples
Embodiment Construction
[0024] In this embodiment, the process is as follows figure 1 As shown, a flow chart of image classification network training based on massive single-category single-frame images. The specific implementation mainly includes the following steps:
[0025] Step (1): Data preparation: Use 1 million single-type and single-face ID card datasets as training dataset 1, and use Webface face dataset as training dataset 2.
[0026] Step (2): Data preprocessing: convert the prepared images of the two face datasets into 128*128 resolution.
[0027] Step (3): Alternate cycle training is based on the image classification network of massive single class single image: Mini-batch is the number of data samples processed in each batch of training, and the present invention adopts that the number of training in each batch is 64, when When the number of training iterations is odd, randomly select 64 pictures from dataset 1 without repetition, and input them into the image classification network. I...
PUM
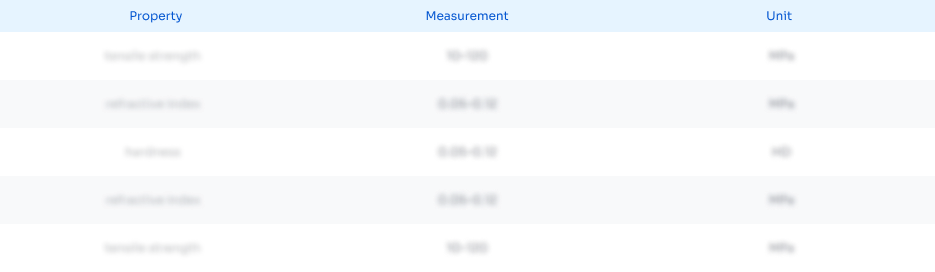
Abstract
Description
Claims
Application Information
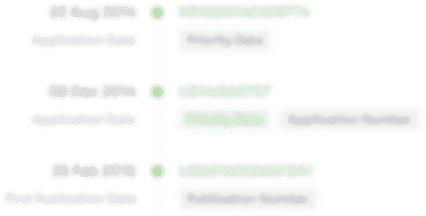
- R&D Engineer
- R&D Manager
- IP Professional
- Industry Leading Data Capabilities
- Powerful AI technology
- Patent DNA Extraction
Browse by: Latest US Patents, China's latest patents, Technical Efficacy Thesaurus, Application Domain, Technology Topic.
© 2024 PatSnap. All rights reserved.Legal|Privacy policy|Modern Slavery Act Transparency Statement|Sitemap