Fault diagnosis method of rotating machinery based on optimized structure convolutional neural network
A convolutional neural network, rotating machinery technology, applied in neural learning methods, biological neural network models, testing of machine/structural components, etc. problems such as low rate, to achieve the effect of convenient deployment and use, avoiding information loss, and less parameters
- Summary
- Abstract
- Description
- Claims
- Application Information
AI Technical Summary
Problems solved by technology
Method used
Image
Examples
Embodiment
[0044] In order to better illustrate the technical scheme and technical effect of the present invention, a specific example is used to analyze and illustrate the working process and technical effect of the present invention. Bearing fault is a typical fault in rotating machinery. Therefore, this example adopts the bearing fault open data of Case Western Reserve University in the United States and the bearing fault data collected by the self-built fault simulation platform to carry out experimental tests. The data used are all dimensional acceleration vibration signal data.
[0045] For the bearing fault data of Western Reserve University, three types of faults are selected: rolling element fault (B), inner ring fault (IR), outer ring fault (OR) and a set of normal data (NR); each fault type is divided into There are 4 kinds of failure degrees, which are 0.18mm, 0.36mm, 0.54mm, and 1mm respectively; the one-dimensional acceleration vibration signals of the above failure modes a...
PUM
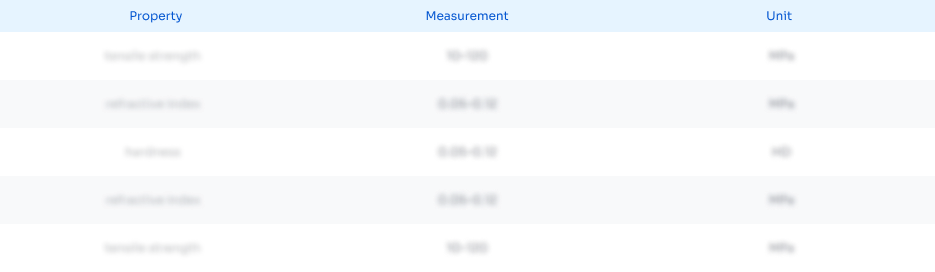
Abstract
Description
Claims
Application Information
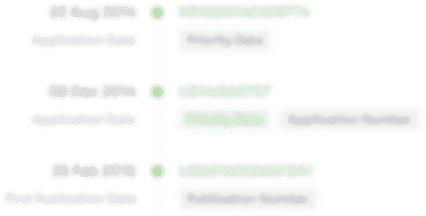
- R&D Engineer
- R&D Manager
- IP Professional
- Industry Leading Data Capabilities
- Powerful AI technology
- Patent DNA Extraction
Browse by: Latest US Patents, China's latest patents, Technical Efficacy Thesaurus, Application Domain, Technology Topic, Popular Technical Reports.
© 2024 PatSnap. All rights reserved.Legal|Privacy policy|Modern Slavery Act Transparency Statement|Sitemap|About US| Contact US: help@patsnap.com