A CT image pulmonary parenchyma three-dimensional semantic segmentation method based on a deep neural network
A deep neural network and CT image technology, applied in image segmentation, the field of three-dimensional semantic segmentation of CT image lung parenchyma based on deep neural network, can solve the problems of noise sensitivity, inability to make full use of the special information of volume data, and the effect is not optimal
- Summary
- Abstract
- Description
- Claims
- Application Information
AI Technical Summary
Problems solved by technology
Method used
Image
Examples
Embodiment Construction
[0060]Below in conjunction with accompanying drawing of description, the embodiment of the present invention is described in detail:
[0061] The offline part is divided into 4 steps:
[0062] Step 1 Preprocessing of the dataset
[0063] The image I is normalized by the following formula using the window width and window level.
[0064]
[0065]
[0066]
[0067] In the formula, Winc is the WindowCenter read from the image, indicating the window level of the image; Winw is the WindowWidth read from the image, indicating the window width of the image; R is the RescaleSlope read from the image, indicating the pixel value RI is the RescaleIntercept read from the image, which represents the scale parameter of the pixel value; i represents each pixel of the image; N is the normalization function.
[0068] Then the images of different sizes are uniformly cropped to a size of 508×508 to form a unified sequence image. Finally, experienced doctors manually label the lung pa...
PUM
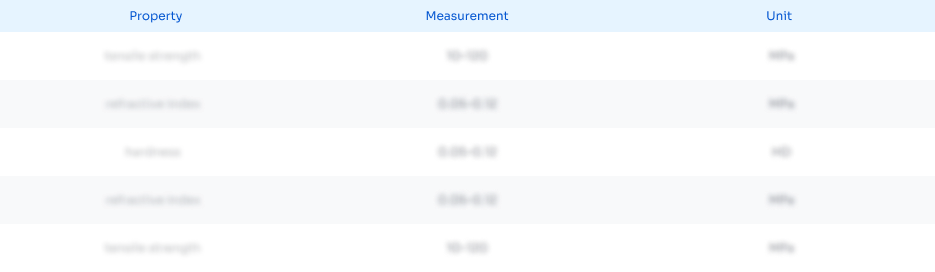
Abstract
Description
Claims
Application Information
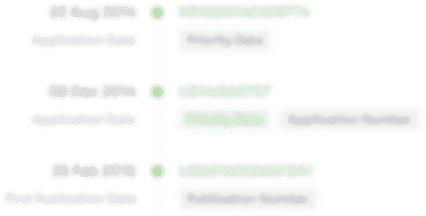
- R&D Engineer
- R&D Manager
- IP Professional
- Industry Leading Data Capabilities
- Powerful AI technology
- Patent DNA Extraction
Browse by: Latest US Patents, China's latest patents, Technical Efficacy Thesaurus, Application Domain, Technology Topic, Popular Technical Reports.
© 2024 PatSnap. All rights reserved.Legal|Privacy policy|Modern Slavery Act Transparency Statement|Sitemap|About US| Contact US: help@patsnap.com