A building energy consumption prediction method based on a recurrent neural network and a multi-task learning model
A cyclic neural network and multi-task learning technology, applied in biological neural network models, prediction, neural architecture, etc., can solve problems such as low accuracy and the inability of unified prediction and prediction process for multiple loads, to simplify the update process, speed up the training speed, The effect of improving forecast accuracy and speed
- Summary
- Abstract
- Description
- Claims
- Application Information
AI Technical Summary
Problems solved by technology
Method used
Image
Examples
Embodiment Construction
[0050] Below in conjunction with accompanying drawing and specific embodiment, the present invention is described in detail, and concrete process structure is as follows image 3 as shown,
[0051] Step 1. Obtain a sample set of building energy consumption data, and use the method of "data averaging at multiple similar time points" to fill in missing data at individual time points. The specific method is as follows:
[0052] Missing data x for a certain type of energy consumption i , let the collection time point be t i , select the time step s, if the time interval unit between sample points is hour, then s is 24 hours, if the time interval unit between sample points is day, then s is 30 days, and so on to determine the size of s . Choose time point as t i The sample points corresponding to ±ns(n=1,2,...), where t i The range of ±ns is within the range of time points corresponding to the first sample point and the last sample point.
[0053] The processed data set struc...
PUM
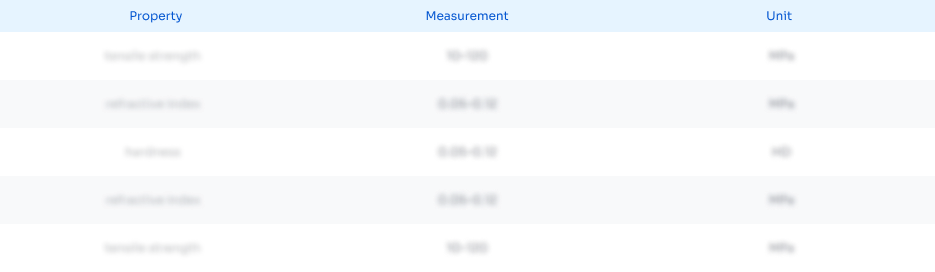
Abstract
Description
Claims
Application Information
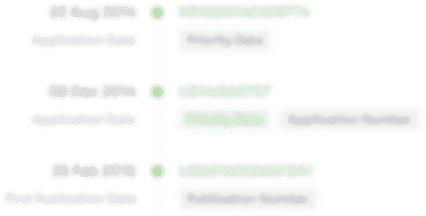
- R&D Engineer
- R&D Manager
- IP Professional
- Industry Leading Data Capabilities
- Powerful AI technology
- Patent DNA Extraction
Browse by: Latest US Patents, China's latest patents, Technical Efficacy Thesaurus, Application Domain, Technology Topic, Popular Technical Reports.
© 2024 PatSnap. All rights reserved.Legal|Privacy policy|Modern Slavery Act Transparency Statement|Sitemap|About US| Contact US: help@patsnap.com