Emotion detection method based on multi-task and multi-label residual neural network
A neural network and detection method technology, applied in the field of service computing, can solve the problems of low neural network accuracy and small amount of available data, and achieve the effect of improving multi-task methods and good versatility
- Summary
- Abstract
- Description
- Claims
- Application Information
AI Technical Summary
Problems solved by technology
Method used
Image
Examples
Embodiment 1
[0050] Below is the specific embodiment of the application of the present invention:
[0051]Collect dataset documents, dataset documents include two datasets: SFEW2.0 dataset and EmotioNet dataset. Among them, the SFEW2.0 data set contains 958 training data, 436 verification data, and 372 test data. These include seven emotion categories. Whereas the EmotioNet dataset includes 25,000 pieces of facial expression data with manual annotations, from which 2,000 pieces of data are annotated with seven emotion categories.
[0052] Executing step 1, the process of labeling the image data is as follows: label the emotion "angry" as "1", label the emotion as "disgust" as "2", and label the emotion as "fear" is "3", the label with the emotion "happy" is marked "4", the label with the emotion "sad" is marked "5", the label with the emotion "surprise" is marked "6", and the label with the emotion is "Neutral" is labeled "7";
[0053] Execute step 2 and use the one-hot tool to convert...
PUM
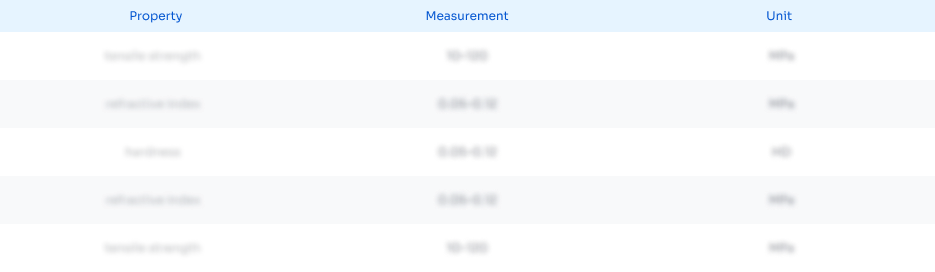
Abstract
Description
Claims
Application Information
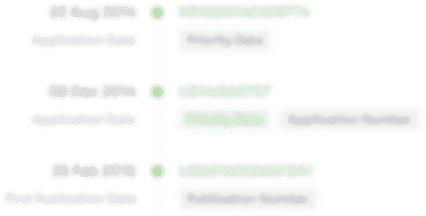
- R&D Engineer
- R&D Manager
- IP Professional
- Industry Leading Data Capabilities
- Powerful AI technology
- Patent DNA Extraction
Browse by: Latest US Patents, China's latest patents, Technical Efficacy Thesaurus, Application Domain, Technology Topic, Popular Technical Reports.
© 2024 PatSnap. All rights reserved.Legal|Privacy policy|Modern Slavery Act Transparency Statement|Sitemap|About US| Contact US: help@patsnap.com