A Learning Control Method for Complex Nonlinear Systems Based on Non-Strictly Repeated Problems
A nonlinear system, learning control technology, applied in the field of learning control of complex nonlinear systems based on non-strictly repetitive problems
- Summary
- Abstract
- Description
- Claims
- Application Information
AI Technical Summary
Problems solved by technology
Method used
Image
Examples
Embodiment 1
[0090] Embodiment one: the system equation of the controlled nonlinear system is as follows:
[0091]
[0092] in, is an unknown parameter that is not strictly repeated, and its change law satisfies the high-order internal model; is a system function vector that satisfies linear growth. unknown parameters Bounded variation in the interval [-1.4,+1.4]; The bounded change interval of is [-3,+3]; The bounded change interval of is [-0.1,+0.1]; the bounded change interval of external disturbance is [-0.1,+0.1]. The system runs iteratively in the discrete time interval {0,1,…,100}.
[0093] The iterative change law of satisfies different high-order internal models respectively, as shown in the following formula:
[0094]
[0095] It can be seen from the above formula that the unknown parameter and respectively satisfy the second-order internal model, and Then satisfy the first-order internal model, that is, the unknown parameter Varies only with time, inde...
Embodiment 2
[0116] Embodiment 2: In order to better investigate the scope of application of the proposed complex nonlinear system learning control method based on non-strictly repetitive problems, considering that strict repetition in the iterative domain is a special case of non-strict repetition, the designed learning control The method is applied to the following control problem of a permanent magnet linear motor:
[0117]
[0118] Among them, v k (t) represents the angular velocity of the mover of the permanent magnet linear motor, and the nonlinear functions of the system are and The iteration domain of unknown parameters of the system is strictly repeated, as follows: θ (1) =0.8237, θ (2) = θ (3) = θ (4) =-0.014, control gain b=0.0014, disturbance d(t)=-0.07sin(0.001πt). The system tracking reference trajectory is where coefficient Randomly takes values in the interval (0,1] as the iteration changes.
[0119] At this time, the boundary of the unknown parameters o...
Embodiment 3
[0121] Embodiment 3: For a single-input single-output system with multiple non-strictly repeated problems, the system equation is considered as follows:
[0122]
[0123] Among them, the unknown parameter θ k (t) changes with the time domain-iterative domain, and the range of change is known, and the change law is the same as that in Embodiment 1 Unknown control gain B(t)=(1+sin(0.5t)), disturbance d(t)=0.1cos(0.05t). System tracking reference trajectory is the same as that in Embodiment 1 The controlled system runs iteratively within a finite time interval t∈{0,1,…,100}.
[0124] According to the formula (17), take the initial value of the learning gain matrix A complex nonlinear system learning control method based on non-strictly repeated problems is run iteratively 100 times in the discrete time interval {0,1,...,100}, and the learning convergence of the maximum absolute value error of state tracking as table 1 and Figure 4 shown.
[0125] Table 1. The maximum...
PUM
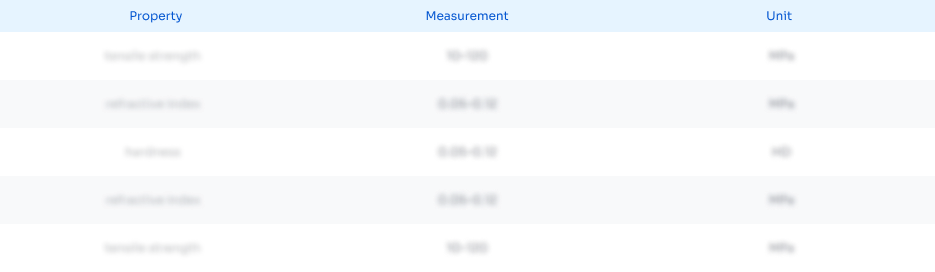
Abstract
Description
Claims
Application Information
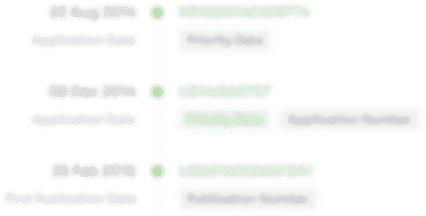
- R&D Engineer
- R&D Manager
- IP Professional
- Industry Leading Data Capabilities
- Powerful AI technology
- Patent DNA Extraction
Browse by: Latest US Patents, China's latest patents, Technical Efficacy Thesaurus, Application Domain, Technology Topic, Popular Technical Reports.
© 2024 PatSnap. All rights reserved.Legal|Privacy policy|Modern Slavery Act Transparency Statement|Sitemap|About US| Contact US: help@patsnap.com