Tunnel lining crack image segmentation method
An image and lining technology, applied in the field of image recognition, can solve problems such as the inability to accurately obtain crack attribute characteristics, achieve the effects of improving recognition accuracy and recognition efficiency, reducing false alarm rate, and avoiding the influence of subjective factors
- Summary
- Abstract
- Description
- Claims
- Application Information
AI Technical Summary
Problems solved by technology
Method used
Image
Examples
Embodiment 1
[0049] Such as figure 1As shown, Embodiment 1 of the present invention provides a method for segmenting tunnel lining crack images, and the method includes the following process steps:
[0050] Step S110: Divide the directly collected original crack image into multiple image blocks, and select the image block with cracks and the corresponding binary image as the training sample set;
[0051] Step S120: De-mean and normalize the image blocks with cracks in the training sample set;
[0052] Step S130: using the convolutional neural network and combining the loss function to train the training sample set after de-mean and normalization processing to obtain a crack segmentation model;
[0053] Step S140: Input the fracture image to be segmented into the fracture segmentation model after demeaning and normalizing, and output the segmentation result image.
[0054] In Embodiment 1 of the present invention, the step S110 specifically includes:
[0055] Each original crack image di...
Embodiment 2
[0077] Such as Figure 5 As shown, the training process and testing process of a deep neural network model for crack region segmentation in tunnel lining images provided by Embodiment 2 of the present invention.
[0078] In the second embodiment of the present invention, a large amount of detailed information needs to be extracted to finely segment the slender crack region. Therefore, the neural network of the present invention incorporates the low-dimensional features (edges, gradients, etc.) extracted during the training process. And high-dimensional features (semantic features of cracks, etc.), through training, the final high-precision segmentation effect is obtained.
[0079] For the directly collected fracture image, its size is 1000×4000 pixels. During the training process of the neural network, the crack image size is relatively large for the input of the network. Each image is divided into 100 sub-blocks of 200×200 pixels, a large number of image blocks with cracks ...
PUM
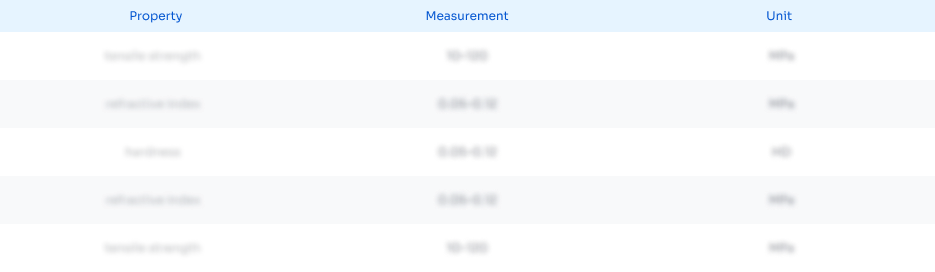
Abstract
Description
Claims
Application Information
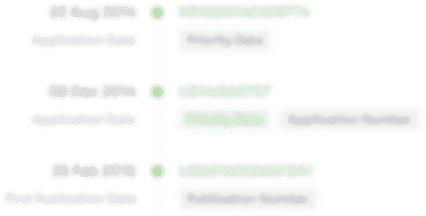
- R&D Engineer
- R&D Manager
- IP Professional
- Industry Leading Data Capabilities
- Powerful AI technology
- Patent DNA Extraction
Browse by: Latest US Patents, China's latest patents, Technical Efficacy Thesaurus, Application Domain, Technology Topic, Popular Technical Reports.
© 2024 PatSnap. All rights reserved.Legal|Privacy policy|Modern Slavery Act Transparency Statement|Sitemap|About US| Contact US: help@patsnap.com