Image fine-grained recognition method based on reinforcement learning strategy
A technology of reinforcement learning and recognition methods, applied in character and pattern recognition, instruments, computer components, etc., can solve problems such as easy confusion, large variance, and many similar features of subcategories
- Summary
- Abstract
- Description
- Claims
- Application Information
AI Technical Summary
Problems solved by technology
Method used
Image
Examples
Embodiment Construction
[0035] Below in conjunction with accompanying drawing and specific embodiment the present invention is described in further detail:
[0036] The present invention provides an image fine-grained recognition method based on a reinforcement learning strategy. For the fine-grained recognition of image subclasses, the existing methods fail to dig out the most representative regions of the image. There is a large inaccuracy on the category. The invention proposes a fine-grained recognition method for mining the most discriminative region of an image on the basis of cross-bilinear features combined with a reinforcement learning strategy.
[0037]Use reinforcement learning Actor-Critic strategy combined with cross bilinear features to mine the most discriminative areas of fine-grained images, and then fuse the original image features to predict fine-grained categories. The method includes the following steps:
[0038] (1) Augment the fine-grained training data. Data augmentation me...
PUM
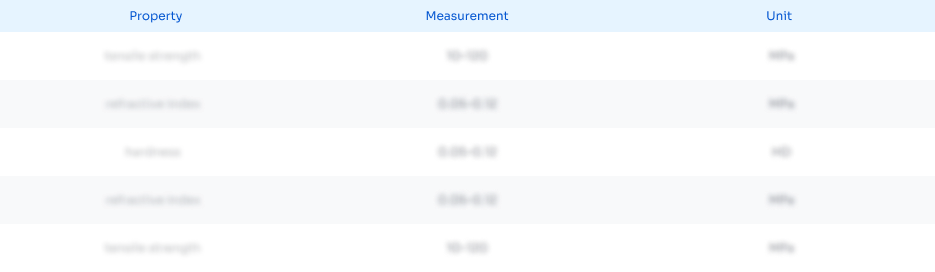
Abstract
Description
Claims
Application Information
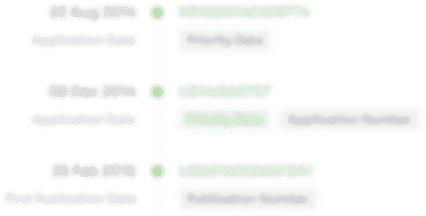
- R&D Engineer
- R&D Manager
- IP Professional
- Industry Leading Data Capabilities
- Powerful AI technology
- Patent DNA Extraction
Browse by: Latest US Patents, China's latest patents, Technical Efficacy Thesaurus, Application Domain, Technology Topic, Popular Technical Reports.
© 2024 PatSnap. All rights reserved.Legal|Privacy policy|Modern Slavery Act Transparency Statement|Sitemap|About US| Contact US: help@patsnap.com