Optimized kernel principal component analysis fault monitoring method based on chaotic particle swarm
A technology of chaotic particle swarm and nuclear principal component analysis, which is applied in the direction of chaotic models, instruments, calculation models, etc., can solve fault monitoring misdetection and missed detection, cannot realize kernel function optimization, cannot calculate data characteristics and monitoring models, etc. question
- Summary
- Abstract
- Description
- Claims
- Application Information
AI Technical Summary
Problems solved by technology
Method used
Image
Examples
Embodiment 1
[0064] This embodiment provides a method for fault monitoring based on chaotic particle swarm optimized kernel principal component analysis, such as Figure 4 as shown, Figure 4 It is a flowchart of another chaotic particle swarm-based optimization kernel principal component analysis fault monitoring method according to Embodiment 1 of the present invention, including the following steps:
[0065] Step S401: Obtain an initial data matrix; the initial data matrix includes a normal data sample matrix and a faulty data sample matrix;
[0066] Step S402: Map the initial data space to the implicit feature space through the nonlinear mapping φ, and perform nonlinear feature transformation in the implicit feature space;
[0067] In the embodiment of the present invention, the specific principle of the nuclear principal component analysis method is:
[0068] Given an initial data matrix X, using M samples and sampling N variables M times, the covariance C can be expressed by the no...
PUM
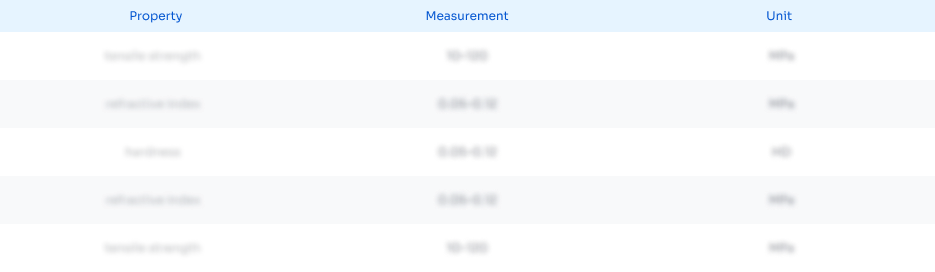
Abstract
Description
Claims
Application Information
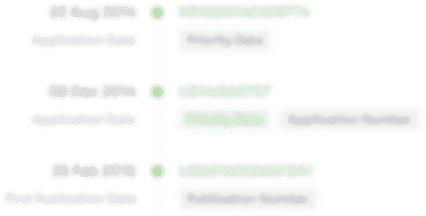
- R&D Engineer
- R&D Manager
- IP Professional
- Industry Leading Data Capabilities
- Powerful AI technology
- Patent DNA Extraction
Browse by: Latest US Patents, China's latest patents, Technical Efficacy Thesaurus, Application Domain, Technology Topic, Popular Technical Reports.
© 2024 PatSnap. All rights reserved.Legal|Privacy policy|Modern Slavery Act Transparency Statement|Sitemap|About US| Contact US: help@patsnap.com