Method for identifying a named entity based on policy value network and tree search
A named entity recognition and tree search technology, applied in the field of information processing, can solve problems such as labor-intensive
- Summary
- Abstract
- Description
- Claims
- Application Information
AI Technical Summary
Problems solved by technology
Method used
Image
Examples
Embodiment Construction
[0019] Next, embodiments of the present invention will be described in more detail.
[0020] First, the first part of the present invention is introduced: Markov decision process modeling.
[0021] Reinforcement learning is another important learning category in machine learning. It mainly uses a trial-and-error mechanism to interact with the environment, and finally achieves the goal of maximizing cumulative rewards. The Markov decision process of reinforcement learning mainly includes five elements: state, action, transition probability, reward and decay factor. figure 1 A schematic diagram of a Markov decision process is given. Suppose X={x 1 ,x 2 ,...,x M} is the word sequence that needs to be marked, Z={z 1 ,z 2 ,…,z M} corresponds to the real label sequence, where M is the length of the sequence, and the design process of the Markov decision process is as follows:
[0022] Step S1: State: Define the state (S) at time t as the following triplet.
[0023]
[00...
PUM
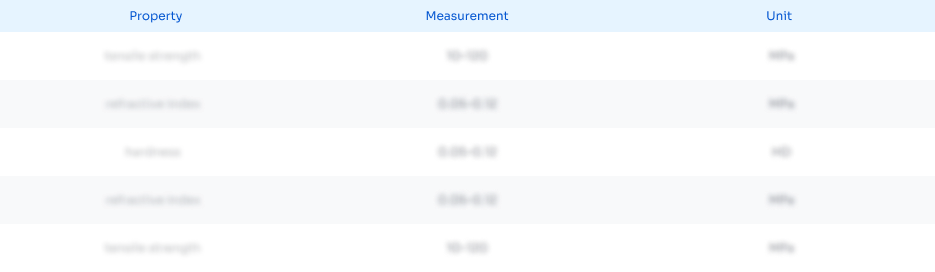
Abstract
Description
Claims
Application Information
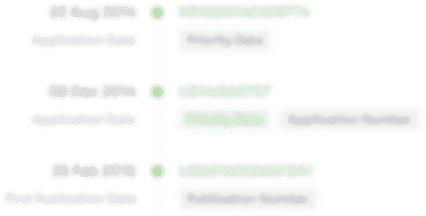
- R&D Engineer
- R&D Manager
- IP Professional
- Industry Leading Data Capabilities
- Powerful AI technology
- Patent DNA Extraction
Browse by: Latest US Patents, China's latest patents, Technical Efficacy Thesaurus, Application Domain, Technology Topic, Popular Technical Reports.
© 2024 PatSnap. All rights reserved.Legal|Privacy policy|Modern Slavery Act Transparency Statement|Sitemap|About US| Contact US: help@patsnap.com