Image data multi-label classification method
A technology of image data and classification method, which is applied in the field of neural network, can solve the problem that the secondary correlation and multiple correlation of labels cannot be extracted at the same time, and achieve good prediction effect
- Summary
- Abstract
- Description
- Claims
- Application Information
AI Technical Summary
Problems solved by technology
Method used
Image
Examples
Embodiment Construction
[0057] The present invention will be further described below in conjunction with drawings and embodiments.
[0058] Embodiments of the present invention are as follows:
[0059] The label set of the specific implementation data set is {plant, bird, person, sky, cloud, grass, mountain}, figure 1 is a sample of this dataset. The input image x of this sample is the pixel point of the image. It can be seen that the label of the sample is {bird, sky, cloud}, and the label of the image is y=[0, 1, 0, 1, 1, 0, 0].
[0060] figure 2 A model structure of the invention is shown.
[0061] step one:
[0062] Obtain the image vector x=[x from the input image 1 , x 2 ,...,x p ] T Get the image feature set V emb ={v 1 x 1 , v 2 x 2 ,...,v p x p}, where x 1 , x 2 ,...,x p respectively represent the gray value of each pixel in the input image, x∈R p , R p Represents the p-dimensional real number space as the input space, p represents the total number of pixels in the inpu...
PUM
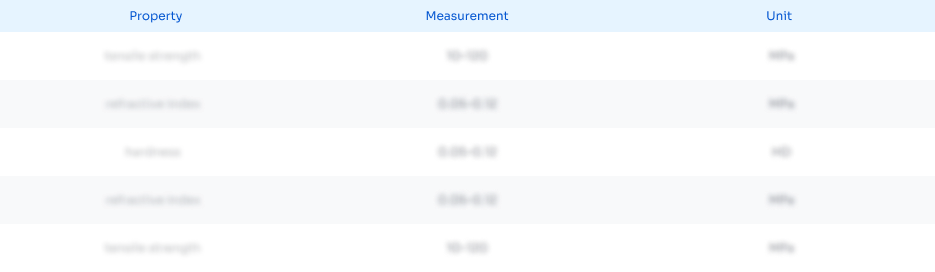
Abstract
Description
Claims
Application Information
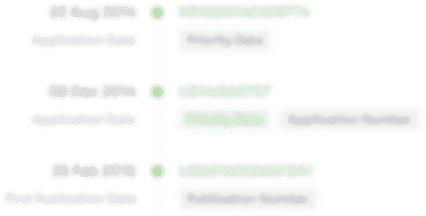
- R&D Engineer
- R&D Manager
- IP Professional
- Industry Leading Data Capabilities
- Powerful AI technology
- Patent DNA Extraction
Browse by: Latest US Patents, China's latest patents, Technical Efficacy Thesaurus, Application Domain, Technology Topic, Popular Technical Reports.
© 2024 PatSnap. All rights reserved.Legal|Privacy policy|Modern Slavery Act Transparency Statement|Sitemap|About US| Contact US: help@patsnap.com