Method for improving product qualification rate based on multi-dimensional decision tree group
A technology of product qualification rate and decision tree, applied in the direction of instruments, data processing applications, resources, etc., can solve the problems of inability to unify, the influence of the qualification rate is large, and the machine parameters are many, so as to reduce the amount of correction, improve the product qualification rate, improve The effect of work efficiency
- Summary
- Abstract
- Description
- Claims
- Application Information
AI Technical Summary
Problems solved by technology
Method used
Examples
Embodiment 1
[0025] Based on the method of improving product qualification rate based on multidimensional decision tree group, the current working conditions are combined with historical data to give suggestions for improving product qualification rate.
[0026] For realizing above-mentioned technical effect, described method comprises:
[0027] S10 collects the basic working conditions that affect the product qualification rate on the production line, and the basic working conditions include equipment parameters and environmental parameters;
[0028] S20 is to construct different decision trees and form a group of decision trees by using production factors as classification standards, wherein the production factors include relatively fixed product categories, models, raw material materials, and the like. Historical data is stored under the decision tree, and the historical data includes historical basic working conditions and one-to-one corresponding product qualification rates.
[0029]...
Embodiment 2
[0033] On the basis of Embodiment 1, the S10 includes S11: find out the main influencing factors in the basic working conditions, that is, calculate the degree of influence of each influencing factor on the product qualification rate and the reliability of the degree of influence. Vectorization and discretization training tools can be used to discretize historical data. Discretization algorithms provide multiple algorithms for selection, such as LBG, K-Means, Mean-Shift, DBSCAN and other clustering algorithms, and then use the information gain rate of information theory Calculate the degree of influence of the basic working conditions on the product qualification rate, and then calculate its reliability according to the TTest algorithm. At the same time, the step S50 includes: the optimization suggestion includes providing the influence degree of a single basic working condition or a combination of basic working conditions in any dimension on the product qualification rate and ...
Embodiment 3
[0036] On the basis of Embodiment 1 or 2, after the decision tree is matched, the current basic working conditions and the actual measured product productivity constitute a dynamic empirical knowledge vector, and the machine learning model iteratively learns the dynamic knowledge vector to support Dynamic growth of decision trees.
[0037] The machine learning model also has a dynamic experience knowledge vector iterative learning function, that is, the machine learning model fine-tunes the value of the basic working conditions on the basis of the existing historical data, and analyzes the influence of the data trend of each basic working condition on the pass rate , learn the fine-tuned product pass rate, so that it can learn a better experience beyond the experience of manual adjustment.
PUM
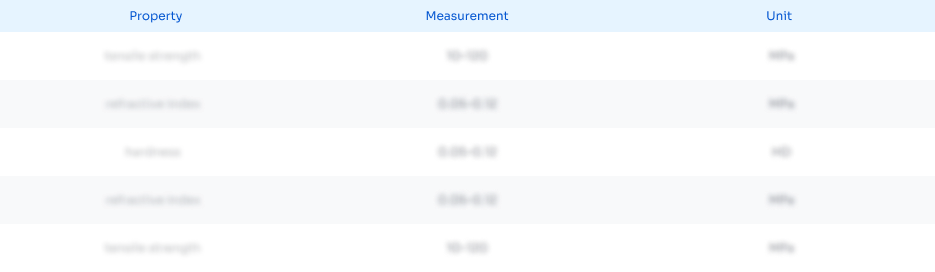
Abstract
Description
Claims
Application Information
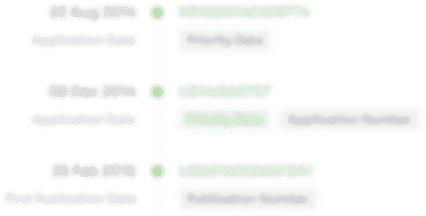
- R&D Engineer
- R&D Manager
- IP Professional
- Industry Leading Data Capabilities
- Powerful AI technology
- Patent DNA Extraction
Browse by: Latest US Patents, China's latest patents, Technical Efficacy Thesaurus, Application Domain, Technology Topic.
© 2024 PatSnap. All rights reserved.Legal|Privacy policy|Modern Slavery Act Transparency Statement|Sitemap