Traffic anomaly detection method, model training method and device
A traffic anomaly and model-determining technology, applied in digital transmission systems, data exchange networks, electrical components, etc., can solve the problem of low accuracy of network traffic data, incompetence of manual work for abnormal detection, and network traffic data distribution that does not obey the normal distribution. and other problems to achieve the effect of improving the accuracy
- Summary
- Abstract
- Description
- Claims
- Application Information
AI Technical Summary
Problems solved by technology
Method used
Image
Examples
Embodiment Construction
[0167] For ease of understanding, several concepts involved in the embodiments of the present application are firstly introduced below.
[0168] 1. A time series is a set of data point sequences arranged in chronological order. Usually the time interval of a set of time series is a constant value, so the time series can be analyzed and processed as discrete time data. Anomaly detection in time series is usually to find data points that deviate from a relatively established pattern or distribution. Time series anomalies include: sudden rise, sudden drop, mean change, etc. Anomaly detection algorithms for time series include algorithms based on statistics and data distribution (N-Sigma), algorithms based on distance / density (local anomaly factor algorithm), isolation forest, and prediction-based algorithms (ARIMA), etc.
[0169] 2. Traffic anomaly detection. Anomaly detection is performed on the traffic data collected from devices or ports in the network. The abnormal detectio...
PUM
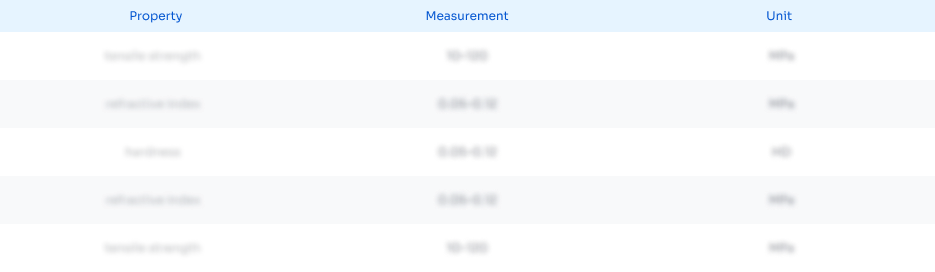
Abstract
Description
Claims
Application Information
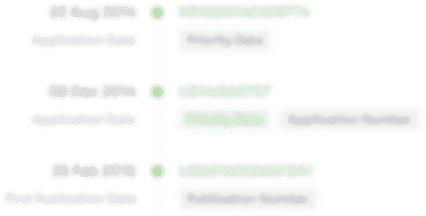
- R&D Engineer
- R&D Manager
- IP Professional
- Industry Leading Data Capabilities
- Powerful AI technology
- Patent DNA Extraction
Browse by: Latest US Patents, China's latest patents, Technical Efficacy Thesaurus, Application Domain, Technology Topic, Popular Technical Reports.
© 2024 PatSnap. All rights reserved.Legal|Privacy policy|Modern Slavery Act Transparency Statement|Sitemap|About US| Contact US: help@patsnap.com