3D point cloud semantic segmentation method based on position attention and auxiliary network
A semantic segmentation and auxiliary network technology, applied in the field of data processing, can solve the problems of weak feature representation, not considering more information at the bottom layer, and low segmentation accuracy, so as to achieve the effect of improving segmentation accuracy
- Summary
- Abstract
- Description
- Claims
- Application Information
AI Technical Summary
Problems solved by technology
Method used
Image
Examples
Embodiment Construction
[0021] The present invention will be further described in detail below in conjunction with the accompanying drawings and specific embodiments.
[0022] refer to figure 1 , the implementation steps of this example include the following.
[0023] Step 1, get training set T and test set V.
[0024] 1.1) Download the training file and test file of 3D point cloud data from ScanNet official website, where the training file contains f 0 point cloud scene, the test file contains f 1 point cloud scene, in this embodiment f 0 =1201, f 1 = 312;
[0025] 1.2) Use the histogram to count all f in the training file 0 The number of each category of the point cloud data of a scene, and calculate the weight w of each category k :
[0026]
[0027] Among them, G k Represent the number of the kth class point cloud data, M represents the number of all point cloud data, L represents the number of segmentation categories, L≥2, L=21 in the present embodiment;
[0028] 1.3) For each scene...
PUM
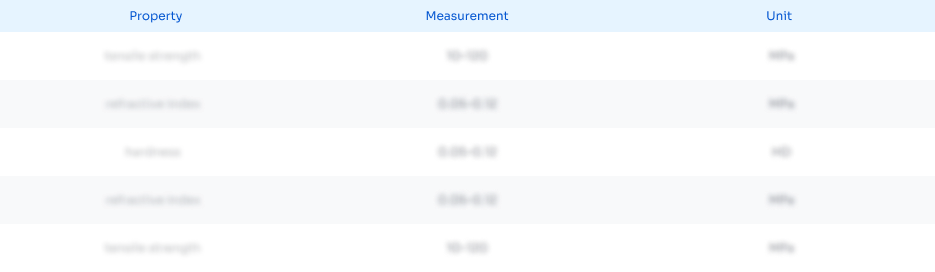
Abstract
Description
Claims
Application Information
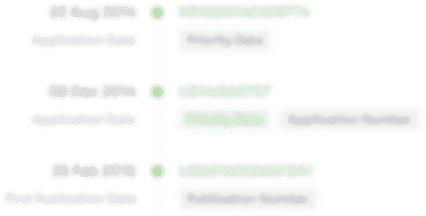
- R&D Engineer
- R&D Manager
- IP Professional
- Industry Leading Data Capabilities
- Powerful AI technology
- Patent DNA Extraction
Browse by: Latest US Patents, China's latest patents, Technical Efficacy Thesaurus, Application Domain, Technology Topic, Popular Technical Reports.
© 2024 PatSnap. All rights reserved.Legal|Privacy policy|Modern Slavery Act Transparency Statement|Sitemap|About US| Contact US: help@patsnap.com