Multi-model integrated target detection method with rich space information
A technology of spatial information and target detection, applied in the image field, can solve the problems of YOLO roughness, single-level frame real-time sacrifice of accuracy, etc., to achieve the effect of verifying effectiveness and improving performance
- Summary
- Abstract
- Description
- Claims
- Application Information
AI Technical Summary
Problems solved by technology
Method used
Image
Examples
Embodiment
[0059] figure 1 It is a flowchart of a multi-model integrated target detection method with rich spatial information in the present invention.
[0060] In this example, if figure 1 As shown, a kind of multi-model integrated target detection method with abundant spatial information of the present invention comprises the following steps:
[0061] S1. Build a network model
[0062] S1.1. Build feature extraction module
[0063] For the feature extraction module, we have chosen 3 modes, built ImageNet pre-trained VGG16 model framework and MobileNet-V1 model framework on Pytorch, and integrated VGG16 and MobileNet-V1 model framework as the feature extraction module;
[0064] S1.2. We built a context module by combining dilated convolution and Incepation-Resnet structure, as shown in Figure 2. The specific operations are as follows:
[0065] Based on the hole convolution and Incepation-Resnet structure, construct three context blocks with the same structure, and then cascade th...
PUM
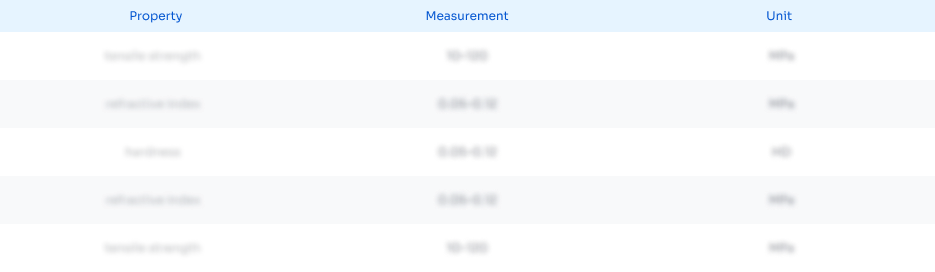
Abstract
Description
Claims
Application Information
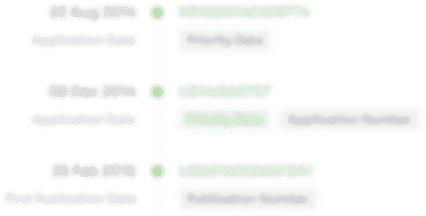
- Generate Ideas
- Intellectual Property
- Life Sciences
- Materials
- Tech Scout
- Unparalleled Data Quality
- Higher Quality Content
- 60% Fewer Hallucinations
Browse by: Latest US Patents, China's latest patents, Technical Efficacy Thesaurus, Application Domain, Technology Topic, Popular Technical Reports.
© 2025 PatSnap. All rights reserved.Legal|Privacy policy|Modern Slavery Act Transparency Statement|Sitemap|About US| Contact US: help@patsnap.com