Genotype predicting method based on deep learning
A prediction method and deep learning technology, applied in the fields of informatics, bioinformatics, instruments, etc., can solve the problems of large computing resources, consumption, and time-consuming, and achieve the effect of solving computing resources, reducing computing time, and saving computing volume.
- Summary
- Abstract
- Description
- Claims
- Application Information
AI Technical Summary
Problems solved by technology
Method used
Image
Examples
Embodiment 1
[0036] Such as Figure 1~2 Shown, a kind of deep learning-based genotype prediction method of the present invention comprises the following steps:
[0037] A: Construct a preliminary training set based on the collected gene fragments;
[0038] B: Perform gene phasing for the preliminary training set, and perform 0, 1, 0.5 encoding on the two haplotypes after gene phasing; divide the encoded data into a training set and a test set according to the ratio of 0.7:0.3;
[0039] C: Construct a neural network model according to the obtained adjacent SNP sites, and use a training set to train the model;
[0040] D: After the test set is processed in steps A and B, it is substituted into the trained neural network model, and the predicted value and model credibility of the test set are obtained;
[0041] E: Substitute the gene sequence that actually needs to be predicted into the model after processing, intercept the sites with a certain degree of reliability as effective prediction,...
Embodiment 2
[0070] Such as Figure 1~4 As shown, based on the method in Example 1, the randomly selected data of 2278 people, that is, 4556 haplotypes were tested, and the results were calculated for comparison.
[0071] The following provides relevant terminology explanations and descriptions:
[0072] Haplotype: Genetically, a combination of alleles that share multiple loci on the same chromosome
[0073] SNP: Single Nucleotide Polymorphism
[0074] Proceed as follows:
[0075] S1: Select the genetic database of 48906 individuals, select 1086 SNP sites as the center, and take 40 sites before and after each as the data set. If the target site is a null value, the data will be discarded. If the adjacent SNP site has a certainty rate of more than 10%, the site will be removed and a new site will be added as input. After cleaning, the effective target site is 896. ; the target site is taken out from the set as the Y value, and the rest as the X value;
[0076] S2: Perform gene phasing ...
PUM
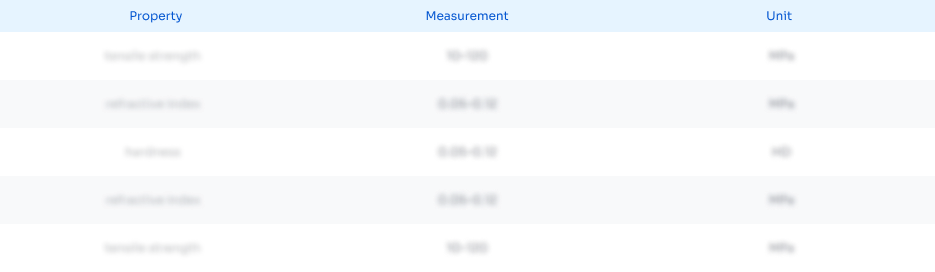
Abstract
Description
Claims
Application Information
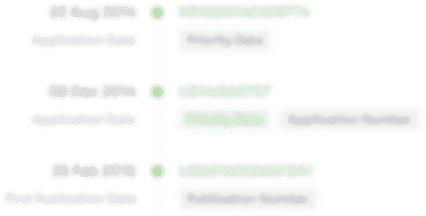
- R&D Engineer
- R&D Manager
- IP Professional
- Industry Leading Data Capabilities
- Powerful AI technology
- Patent DNA Extraction
Browse by: Latest US Patents, China's latest patents, Technical Efficacy Thesaurus, Application Domain, Technology Topic.
© 2024 PatSnap. All rights reserved.Legal|Privacy policy|Modern Slavery Act Transparency Statement|Sitemap