Article recommendation method based on hierarchical multi-granularity matrix decomposition
A matrix decomposition and multi-granularity technology, applied in the field of recommendation, can solve problems such as ignoring information loss, and achieve the effect of accurate preference expression
- Summary
- Abstract
- Description
- Claims
- Application Information
AI Technical Summary
Problems solved by technology
Method used
Image
Examples
Embodiment
[0089] data set:
[0090] The experiment of this embodiment uses three data sets, namely MovieLens-100k, MovieLens-1m and MovieLens-HetRec. Among them, MovieLens-100k and MovieLens-1m are two widely used data sets about movie ratings; MovieLens-100k is a smaller movie rating data set, which includes 100,000 rating data of 1682 movies from 943 users, The MovieLens-1m dataset includes 1,000,209 rating data for 3,706 movies from 6,040 users. In addition, MovieLens-HetRec is a rating data set from MovieLens and IMDB (InternetMovie Database) websites, including 855,598 rating data from 2,113 users on 10,109 movies. The specific statistical information of each data set is shown in Table 1.
[0091] Table 1 Statistics of the dataset
[0092]
[0093] Indicators for comparison:
[0094] Two widely used evaluation metrics for rating prediction tasks are Mean Absolute Error (MAE) and Root Mean Squared Error (RMSE). For any user u and item i in the test set, suppose the actual ra...
PUM
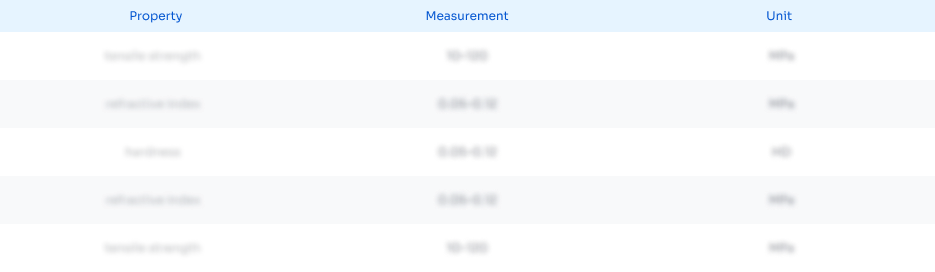
Abstract
Description
Claims
Application Information
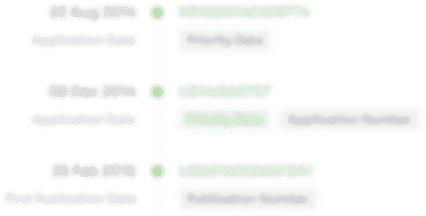
- R&D Engineer
- R&D Manager
- IP Professional
- Industry Leading Data Capabilities
- Powerful AI technology
- Patent DNA Extraction
Browse by: Latest US Patents, China's latest patents, Technical Efficacy Thesaurus, Application Domain, Technology Topic, Popular Technical Reports.
© 2024 PatSnap. All rights reserved.Legal|Privacy policy|Modern Slavery Act Transparency Statement|Sitemap|About US| Contact US: help@patsnap.com