A fall detection method based on video joints and hybrid classifier
A hybrid classifier and detection method technology, applied in the field of fall detection based on video joints and hybrid classifiers, can solve the problems of difficult to reduce detection time consumption, complex models, simple models, etc., to achieve reduced detection time consumption and high accuracy , the effect of reducing the accuracy
- Summary
- Abstract
- Description
- Claims
- Application Information
AI Technical Summary
Problems solved by technology
Method used
Image
Examples
Embodiment 2
[0087] like image 3 As shown, a fall detection method based on video joints and hybrid classifiers differs from Embodiment 1 in that:
[0088] In step 2, instead of performing Gaussian denoising processing on each frame of the detected video clip, grayscale processing is performed.
[0089] In step 6, the secondary classifier is different from that in Embodiment 1. The secondary classifier in this implementation is a multi-scale convolutional neural network (referred to as MultiCNN), including a convolutional layer connected by activation function Relu in turn. , a pooling layer, and three fully connected layers. In the convolution mode of the convolutional neural network, the padding parameter is set to 'valid'. Four convolution kernels with scales of 3×3, 5×5, 7×7, and 9×9 are set in the convolutional layer; Four pooling operators of 2×1; 3×3, 5×5, 7×7, 9×9 convolution kernels and 8×1, 6×1, 4×1, 2×1 pooling operators Subs are connected correspondingly. The stride of th...
PUM
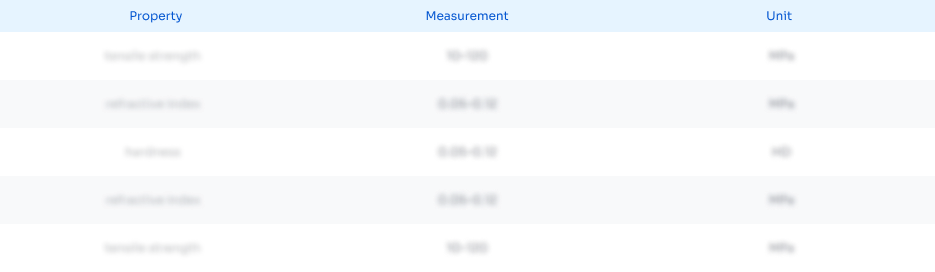
Abstract
Description
Claims
Application Information
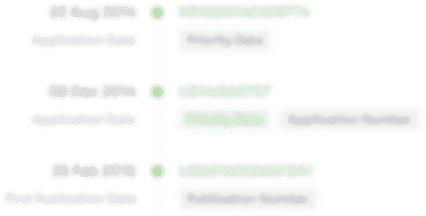
- Generate Ideas
- Intellectual Property
- Life Sciences
- Materials
- Tech Scout
- Unparalleled Data Quality
- Higher Quality Content
- 60% Fewer Hallucinations
Browse by: Latest US Patents, China's latest patents, Technical Efficacy Thesaurus, Application Domain, Technology Topic, Popular Technical Reports.
© 2025 PatSnap. All rights reserved.Legal|Privacy policy|Modern Slavery Act Transparency Statement|Sitemap|About US| Contact US: help@patsnap.com