Probability matrix decomposition cold start recommendation method fusing attributes and semantics
A probabilistic matrix decomposition and recommendation method technology, which is applied in the field of probabilistic matrix decomposition cold start recommendation that integrates attributes and semantics, and can solve problems such as poor scalability, inability to solve cold start, and lack of semantic interpretation.
- Summary
- Abstract
- Description
- Claims
- Application Information
AI Technical Summary
Problems solved by technology
Method used
Image
Examples
Embodiment Construction
[0064] The present invention will be further described below in conjunction with the accompanying drawings.
[0065] refer to Figure 1 ~ Figure 3 , a probabilistic matrix decomposition cold-start recommendation method that combines attributes and semantics. First, user attribute information, item attribute information, item text information, and user rating information are extracted from the database, and linear regression is used to model attribute information and semantic information to predict potential features, and use the predicted value as the prior probability of probability decomposition, so as to realize the fusion of attribute information and semantic information into the probability decomposition of scoring matrix, the method includes the following steps:
[0066] Step 1. Collect a large amount of user and item data, including user attribute information, item attribute information, user rating information on the item and item content text information, and build a ...
PUM
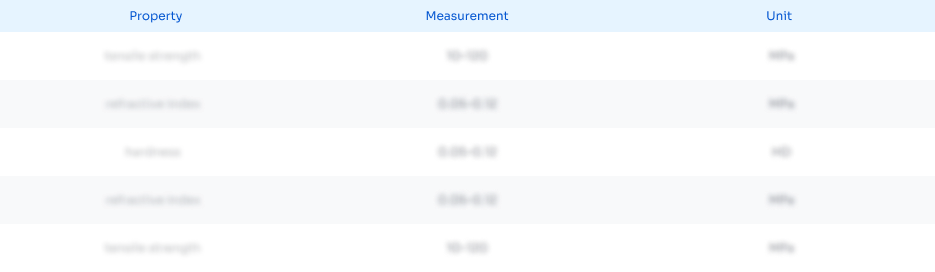
Abstract
Description
Claims
Application Information
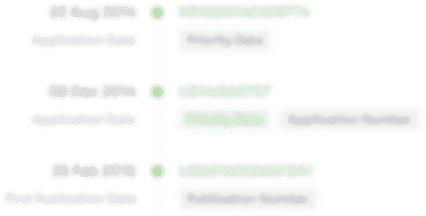
- R&D Engineer
- R&D Manager
- IP Professional
- Industry Leading Data Capabilities
- Powerful AI technology
- Patent DNA Extraction
Browse by: Latest US Patents, China's latest patents, Technical Efficacy Thesaurus, Application Domain, Technology Topic, Popular Technical Reports.
© 2024 PatSnap. All rights reserved.Legal|Privacy policy|Modern Slavery Act Transparency Statement|Sitemap|About US| Contact US: help@patsnap.com