Electroencephalogram classification method based on attention mechanism and convolutional neural network
A technology of convolutional neural network and EEG signal, which is applied in the field of EEG signal classification based on attention mechanism and convolutional neural network, can solve the problem of lack of universality, different classification effects, and insufficient accuracy of EEG signal classification. Advanced problems, to achieve the effect of improving the accuracy of classification
- Summary
- Abstract
- Description
- Claims
- Application Information
AI Technical Summary
Problems solved by technology
Method used
Image
Examples
Embodiment Construction
[0028] see Figure 4 , the present invention a kind of EEG signal classification method based on attention mechanism and convolutional neural network, comprises the following steps:
[0029] S1, preprocessing, assume that the EEG signals of n channels are collected as X n (t), for EEG signals in normal state and abnormal state, intercept a piece of EEG signal every 1-2 s as a classification sample x n (t), in order to ensure that the classifier will not overfit one of the classes, the ratio of the number of samples of the two classes in the experiment is 1:1;
[0030] EEG signals are a non-invasive tool for measuring the electrical activity of the brain, which contains a wealth of information about brain function. An abnormal state characterized by the sudden appearance of abnormal electrical activity in some or the entire brain region, resulting in transient dysfunction of the central nervous system; includes spikes, sharp waves, sharp-slow complexes, sharp-slow complexes, ...
PUM
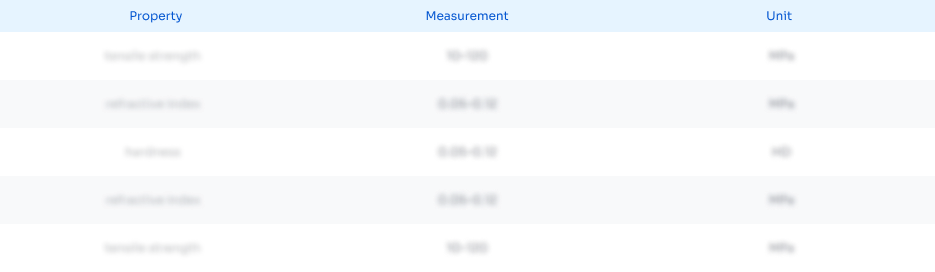
Abstract
Description
Claims
Application Information
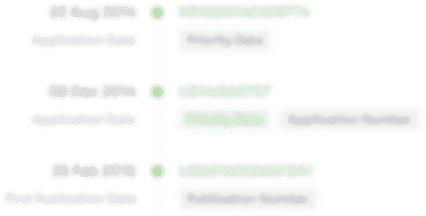
- R&D
- Intellectual Property
- Life Sciences
- Materials
- Tech Scout
- Unparalleled Data Quality
- Higher Quality Content
- 60% Fewer Hallucinations
Browse by: Latest US Patents, China's latest patents, Technical Efficacy Thesaurus, Application Domain, Technology Topic, Popular Technical Reports.
© 2025 PatSnap. All rights reserved.Legal|Privacy policy|Modern Slavery Act Transparency Statement|Sitemap|About US| Contact US: help@patsnap.com