Rolling bearing fault analysis based on CNN and LSTM
A rolling bearing and fault classification technology, applied in the testing of mechanical components, character and pattern recognition, pattern recognition in signals, etc., can solve problems such as gradient disappearance
- Summary
- Abstract
- Description
- Claims
- Application Information
AI Technical Summary
Problems solved by technology
Method used
Image
Examples
Embodiment Construction
[0040] figure 1 It is a schematic flowchart of a rolling bearing fault analysis method based on CNN and LSTM according to an embodiment of the present application. see figure 1 It is known that a CNN and LSTM-based rolling bearing fault analysis method and system provided in the embodiment of the present application may include:
[0041] Step S1: Denoise the processed vibration data using a Butterworth Filter, and perform Fast Fourier Transform (FFT) to convert the preprocessed time-domain signal into a frequency-domain signal;
[0042] Step S2: using CNN network for learning to obtain the image features of the time domain map and the frequency domain map;
[0043] Step S3: performing image feature fusion through the add layer;
[0044]Step S4: Input the fused image features obtained by the add layer into the LSTM network, and further learn the time series features contained in the features through the LSTM network;
[0045] Step S5: realize the classification function thr...
PUM
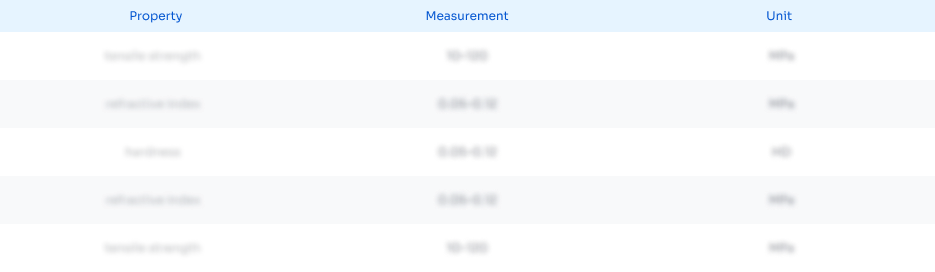
Abstract
Description
Claims
Application Information
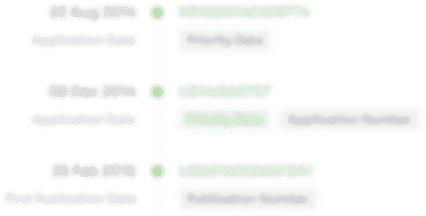
- R&D
- Intellectual Property
- Life Sciences
- Materials
- Tech Scout
- Unparalleled Data Quality
- Higher Quality Content
- 60% Fewer Hallucinations
Browse by: Latest US Patents, China's latest patents, Technical Efficacy Thesaurus, Application Domain, Technology Topic, Popular Technical Reports.
© 2025 PatSnap. All rights reserved.Legal|Privacy policy|Modern Slavery Act Transparency Statement|Sitemap|About US| Contact US: help@patsnap.com