Log sequence anomaly detection framework based on nLSTM (Non-Log Sequence Transfer Module)-self attention
An anomaly detection and logging technology, applied in the field of network security, can solve problems such as insufficient performance characteristics and insufficient processing capabilities, and achieve long-term dependence and significant effects
- Summary
- Abstract
- Description
- Claims
- Application Information
AI Technical Summary
Problems solved by technology
Method used
Image
Examples
Embodiment Construction
[0037] In order to make the purpose, content, and advantages of the present invention clearer, the specific implementation manners of the present invention will be further described in detail below in conjunction with the accompanying drawings and embodiments.
[0038] figure 1 Shown is a schematic diagram of the log sequence anomaly detection framework based on nLSTM-self attention, such as figure 1 As shown, the log sequence anomaly detection framework includes: a training phase and a detection phase. Wherein, the training phase includes: assuming that a log file contains k log templates E={e 1 ,e 2 L e k}, the input of the training phase is a sequence of log templates, a log sequence l of length h t-h ,... l t-2 , l t-1 The log template contained in l i ∈E, t-h≤i≤t-1, and the number of log templates in a sequence|l t-h ,... l t-2 , l t-1 |=m≤h. In order to facilitate data processing, firstly, each log template corresponds to a template number, and a log template ...
PUM
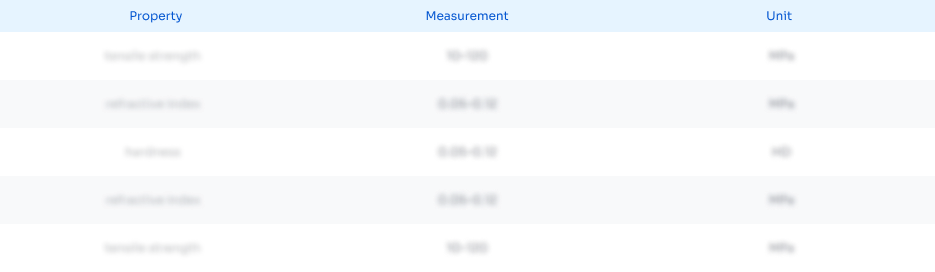
Abstract
Description
Claims
Application Information
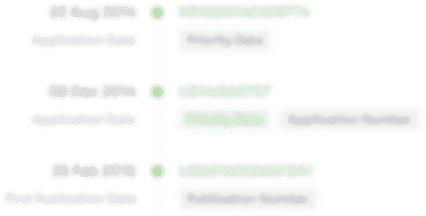
- R&D Engineer
- R&D Manager
- IP Professional
- Industry Leading Data Capabilities
- Powerful AI technology
- Patent DNA Extraction
Browse by: Latest US Patents, China's latest patents, Technical Efficacy Thesaurus, Application Domain, Technology Topic, Popular Technical Reports.
© 2024 PatSnap. All rights reserved.Legal|Privacy policy|Modern Slavery Act Transparency Statement|Sitemap|About US| Contact US: help@patsnap.com